Addressing Challenges In Automated Visual Inspection Of Lyophilized Vials
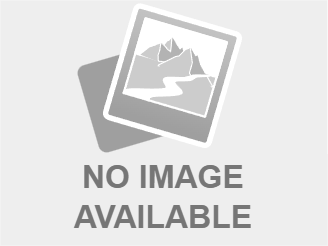
Table of Contents
Challenges in Image Acquisition and Preprocessing
The initial stages of automated visual inspection—image acquisition and preprocessing—present several obstacles. Effective defect detection hinges on acquiring high-quality images that accurately represent the vial’s condition.
Lighting and Shadowing
Non-uniform lighting is a major problem. Shadows obscure defects, hindering accurate detection.
- Variations in vial orientation: Slight changes in vial positioning during inspection lead to inconsistent lighting, creating problematic shadows.
- Surface reflections: The glass surface of the vial can reflect light, further complicating image analysis and masking subtle defects.
- Solutions: Advanced lighting techniques are crucial. Structured lighting, using precisely controlled light patterns, minimizes shadowing. Multi-spectral imaging, capturing images across multiple wavelengths, can also improve contrast and reveal defects otherwise hidden by shadows or reflections. Careful consideration of lighting angles and intensity is paramount for optimal image acquisition.
Particle Detection and Differentiation
Distinguishing between genuine defects (particulate matter, cracks) and harmless variations (lyophilization process artifacts) is critical.
- Image segmentation and classification algorithms: Sophisticated algorithms are necessary to segment the image into relevant regions and classify these regions as defects or non-defects.
- Training data quality: The accuracy of these algorithms heavily depends on the quality and quantity of training data used. A diverse dataset representing various vial conditions and defects is vital.
- Advanced AI techniques: Deep learning models, a subset of AI, excel at learning complex patterns and can significantly improve the discrimination between genuine defects and harmless variations. This contributes to a more precise automated visual inspection process.
Variability in Vial Appearance
Differences in fill levels, stopper position, and lyophilized cake morphology introduce significant variability.
- Robust algorithms: Inspection algorithms must account for this natural variability in vial appearance to avoid false positives or negatives. Adaptive algorithms that learn and adjust to these variations are highly beneficial.
- Calibration and standardization: Careful calibration and standardization of inspection parameters are essential to ensure consistent performance across different batches and vials.
- Data augmentation techniques: Augmenting the training dataset with artificially generated variations can enhance the robustness of the algorithms against real-world variability.
Challenges in Defect Detection and Classification
Even with high-quality images, accurate defect detection and classification remain challenging.
Defining Acceptable Defect Limits
Establishing clear criteria for acceptable versus unacceptable defects is critical for pharmaceutical quality control compliance.
- Collaboration: Close collaboration between quality control specialists and automation engineers is essential to define meaningful and actionable defect criteria.
- Statistical process control (SPC): SPC methods help define acceptable limits based on historical data and process variation. This ensures that the automated visual inspection system aligns with established quality standards.
- Validation and verification: Rigorous validation and verification processes are needed to ensure the automated system's compliance with regulatory guidelines (e.g., FDA, EMA).
Accuracy and Precision of Defect Detection
Minimizing false positives (incorrectly identifying non-defects as defects) and false negatives (missing actual defects) is paramount.
- Advanced image analysis: Employing advanced image analysis algorithms, such as those incorporating machine learning, can drastically improve the accuracy and precision of defect detection.
- Regular system calibration and maintenance: Preventative maintenance and regular recalibration are critical for maintaining the system's accuracy over time.
- Performance monitoring: Continuous performance monitoring and improvement measures, such as implementing feedback loops, are vital for ensuring long-term reliability.
Integration with Existing Quality Control Systems
Seamless integration with existing quality control systems (e.g., LIMS, ERP) is necessary for efficient data management and reporting.
- Data formats and communication protocols: Compatibility with existing systems' data formats and communication protocols is crucial for easy data exchange.
- Data security and integrity: Robust security measures must be implemented to ensure data integrity and protect sensitive information.
- Regulatory compliance: The entire system must be validated and compliant with relevant regulatory standards for pharmaceutical manufacturing.
Addressing the Challenges: Technological Advancements and Best Practices
Overcoming these challenges requires a multifaceted approach incorporating advanced technologies and best practices.
Advanced Machine Vision Systems
High-resolution cameras, combined with sophisticated lighting techniques (as discussed earlier), form the foundation of a successful AVI system. These provide the detailed image data necessary for accurate analysis.
Artificial Intelligence and Machine Learning
AI and ML algorithms, particularly deep learning models, are vital for classifying complex defects and adapting to variations in vial appearance. These algorithms can learn from large datasets and improve their accuracy over time.
Data Management and Analytics
Implementing robust data management systems and utilizing advanced analytics enables a deeper understanding of the inspection process and allows for optimization of inspection parameters. This data-driven approach is key to continuously improving the performance of the AVI system.
Conclusion
Automated visual inspection of lyophilized vials offers significant advantages in efficiency and accuracy for pharmaceutical quality control. However, challenges related to image acquisition, defect detection, and system integration require careful consideration. By leveraging advanced technologies such as AI, machine learning, and improved image analysis techniques, the pharmaceutical industry can overcome these challenges and achieve reliable and efficient automated visual inspection. Investing in robust and validated automated visual inspection systems is crucial for ensuring the quality and safety of lyophilized pharmaceuticals. Embrace the future of pharmaceutical quality control by implementing advanced solutions for automated visual inspection of lyophilized vials.
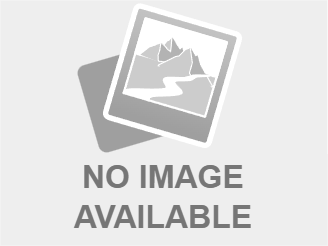
Featured Posts
-
Anunoby Con 27 Puntos Victoria De Knicks Sobre Sixers Su Novena Derrota
May 11, 2025 -
East Tennessee History Center Showcases Baseball History Before Covenant Health Park Opening
May 11, 2025 -
Golf News Mc Ilroy Lowry Team For Zurich Classic
May 11, 2025 -
Ottawa First Of Its Kind 10 Year Deal With Indigenous Capital Group
May 11, 2025 -
Muhammad And Della Maddalenas Heated Exchange At Ufc 315 Press Conference
May 11, 2025