Analyzing The I/O And Io Strategies Of Google And OpenAI
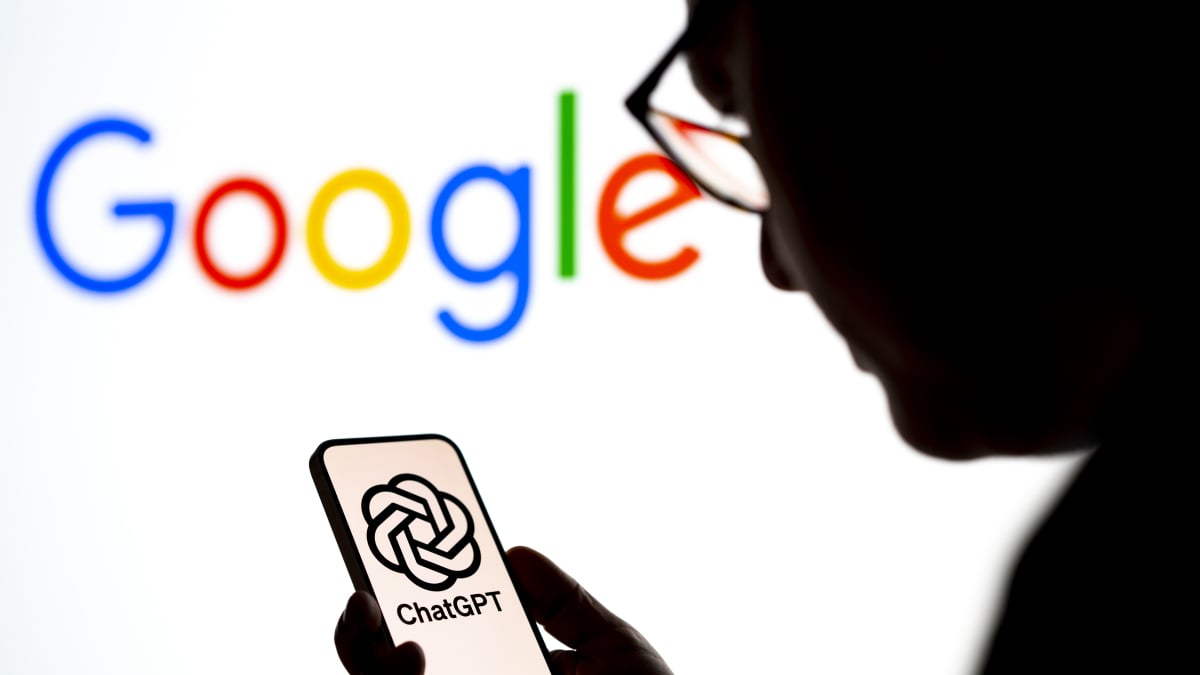
Table of Contents
Google's I/O and IO Approach: A Multifaceted Giant
Google's I/O and IO strategies are characterized by their massive scale and data-centric approach. This multifaceted giant leverages its vast resources to create seamless user experiences across various platforms.
Google's Data-Centric I/O
Google's I/O hinges on its unparalleled ability to collect, process, and utilize massive datasets. This data-centric approach fuels its search algorithms, personalized recommendations, and the training of sophisticated AI models.
-
Impact on Search: Google Search's I/O relies on user queries as input and delivers highly relevant search results as output, constantly learning and adapting based on user interactions and data analysis.
-
Personalized Experiences: Google's services, such as Gmail and YouTube, use user data to personalize experiences, offering tailored recommendations and optimizing user interfaces. This involves sophisticated I/O processes analyzing user behavior and preferences.
-
AI Model Training: Google's vast datasets are crucial for training powerful AI models across various domains, from natural language processing to computer vision. This data-driven approach defines Google's I/O strategy in the AI space.
-
Specific I/O Mechanisms:
- User queries (input) processed through complex algorithms (processing) resulting in search results, personalized ads, and knowledge graph information (output).
- User interactions with Google Assistant (input), processed through natural language understanding models (processing) generating responses and completing tasks (output).
- Location data from Google Maps (input) analyzed to provide real-time traffic updates, navigation instructions, and business information (output).
Google's Cloud-Based IO Infrastructure
Google's cloud-based infrastructure, Google Cloud Platform (GCP), plays a vital role in enabling scalable I/O operations for its services and for external developers. This robust IO infrastructure is essential for handling the massive data flows associated with Google's AI models and applications.
- Scalable AI Model Deployment: GCP provides the computing power and storage capacity needed to deploy and manage large-scale AI models efficiently, optimizing I/O performance and reducing latency.
- Google Kubernetes Engine (GKE): GKE simplifies the deployment, scaling, and management of containerized applications, optimizing resource utilization and improving I/O efficiency. Its robust management of containers is critical to handling complex I/O demands.
- Advantages of GCP's I/O: High scalability, reliability, security, and a comprehensive suite of tools for managing data and applications.
- Disadvantages of GCP's I/O: Cost can be a significant factor for smaller organizations, and the complexity of the platform might present a learning curve for some users.
OpenAI's I/O and IO Approach: Focusing on Model Interaction
OpenAI’s I/O and IO strategies are primarily focused on providing accessible and powerful AI models through APIs and fostering advancements through extensive research.
OpenAI's API-Driven I/O
OpenAI's primary I/O mechanism is its APIs, which enable developers to integrate its AI models (like GPT-3, DALL-E) into their applications. This approach democratizes access to advanced AI capabilities.
- Developer Accessibility: OpenAI's APIs allow developers with varying levels of expertise to leverage powerful AI models without needing deep knowledge of underlying algorithms.
- ChatGPT and DALL-E 2: These models exemplify OpenAI's API-driven I/O strategy, facilitating user interaction through natural language prompts and generating text or images in response.
- Advantages of API-based I/O: Ease of integration, scalability, and rapid prototyping capabilities.
- Limitations of API-based I/O: Dependency on API availability and potential cost implications for high usage.
OpenAI's Research-Focused IO
OpenAI's commitment to research is a defining aspect of its IO strategy. Continuous improvement in model capabilities and I/O efficiency drives innovation.
- Reinforcement Learning from Human Feedback (RLHF): OpenAI heavily utilizes RLHF to align its models with human preferences and improve their I/O performance, making interactions more natural and effective.
- Prompt Engineering: Research in prompt engineering focuses on optimizing user inputs to elicit desired outputs, improving the overall I/O effectiveness of AI models.
- Responsible AI Development: OpenAI emphasizes responsible AI development, integrating safety and ethical considerations into its I/O design, mitigating potential risks associated with powerful AI models.
Comparing and Contrasting Google and OpenAI's I/O and IO Strategies
Feature | OpenAI | |
---|---|---|
Primary Focus | Data-centric, large-scale applications | Model interaction, API accessibility |
I/O Mechanism | Diverse, integrated services | Primarily API-driven |
Infrastructure | Google Cloud Platform (GCP) | Cloud-based, but less emphasis on platform |
Data Usage | Massive datasets for training & services | Focus on curated datasets for model training |
Development | Product-focused, user experience | Research-focused, model capabilities |
Google's strategy emphasizes comprehensive services built upon a vast data infrastructure, whereas OpenAI prioritizes providing accessible and powerful AI models through APIs, fueling research and development in AI capabilities. Google's strength lies in its integrated ecosystem and massive data, while OpenAI’s advantage is the ease of access and model innovation its APIs provide.
Conclusion: Understanding the Future of I/O and IO in AI
Google and OpenAI represent distinct yet influential approaches to I/O and IO in the realm of artificial intelligence. Google's data-centric, integrated approach focuses on building large-scale applications, while OpenAI prioritizes model accessibility and research-driven innovation through APIs. Understanding these differing strategies is critical for developers, researchers, and users alike. Future trends likely include a blurring of these lines, with greater emphasis on efficient and ethical I/O processes, enhanced model interpretability, and further integration of AI into our daily lives. Deepen your understanding of the evolving landscape of I/O and IO strategies by exploring relevant research papers and continue the discussion by sharing your insights in the comments below.
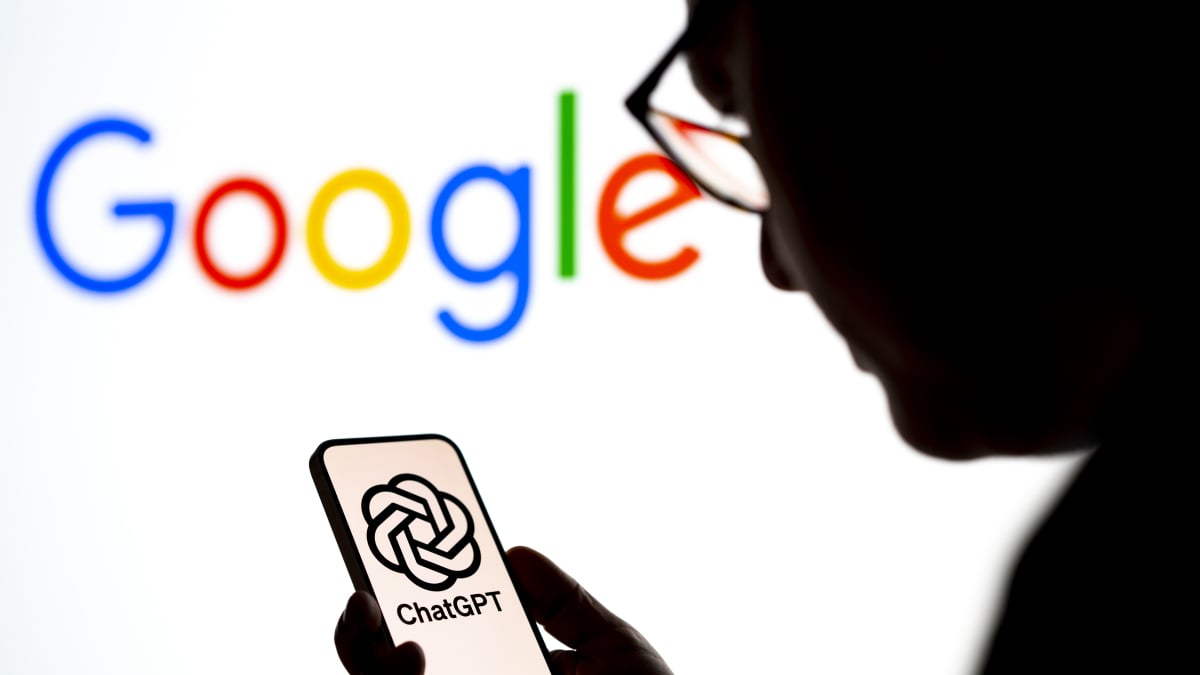
Featured Posts
-
Carolina Country Music Fest 2025 Sells Out What This Means For Fans
May 25, 2025 -
What Is A Flash Flood Understanding Flood Warnings And Alerts
May 25, 2025 -
Oleg Basilashvili Test Na Znanie Ego Filmografii
May 25, 2025 -
Trump E I Dazi Del 20 Analisi Dell Effetto Sul Mercato Della Moda
May 25, 2025 -
Claire Williams And George Russell A Complex Professional Relationship
May 25, 2025
Latest Posts
-
Zheng Qinwens Semifinal Berth At The Italian Open
May 25, 2025 -
Italian Open Zheng Qinwen Reaches Semifinals
May 25, 2025 -
Zheng Qinwens Italian Open Semifinal Run A Breakthrough Performance
May 25, 2025 -
Italian Open Semifinals Gauff Eliminates Zheng In Three Set Battle
May 25, 2025 -
Zheng Eliminated By Gauff In Italian Open Semifinal
May 25, 2025