Big Rig ROCK Report 3.12 | Laser 101.7: Data Analysis And Interpretation
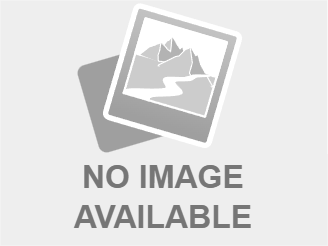
Table of Contents
Understanding the Big Rig ROCK Report 3.12 Dataset
Data Sources and Collection Methods
The Big Rig ROCK Report 3.12 compiles data from various sources to provide a comprehensive overview of fleet performance. This data is typically collected through:
- GPS Tracking Devices: Providing real-time location data, speed, mileage, and route information.
- Electronic Logging Devices (ELDs): Recording driver hours of service (HOS) compliance, driving time, and breaks.
- Vehicle Telematics Systems: Gathering data on engine performance, fuel consumption, idle time, and maintenance alerts.
- Driver Logs and Maintenance Records: Supplementing the automated data with manual entries.
The data points within the report are crucial for understanding various aspects of your fleet's operation. These include:
- Fuel Efficiency (mpg): A key indicator of operational costs.
- Mileage: Total distance covered by each vehicle.
- Idle Time: Time spent with the engine running but not moving, a significant factor in fuel consumption.
- Maintenance Records: Tracking repairs, preventative maintenance, and downtime.
It's crucial to acknowledge potential limitations in data quality. Inaccurate data entry, sensor malfunctions, and incomplete records can significantly impact the reliability of your analysis. Therefore, proper data cleaning and validation are essential steps.
Key Metrics and Variables
The Big Rig ROCK Report 3.12 features several key performance indicators (KPIs) that are essential for effective fleet management. Within the Laser 101.7 data subset, you'll likely find metrics like:
- Fuel Consumption per Mile: Directly reflecting fuel efficiency and cost.
- Average Speed: Indicating potential route optimization opportunities.
- Engine Hours: Helping schedule preventative maintenance and predict potential failures.
- Hard Braking and Acceleration Events: Identifying driver behavior that impacts safety and fuel economy.
Understanding the significance of each KPI allows you to pinpoint areas for improvement. For instance, consistently high idle time in Laser 101.7 data could indicate the need for driver training or operational adjustments.
Data Cleaning and Preprocessing
Before diving into analysis, data cleaning is crucial for reliable results. This involves:
- Handling Missing Values: Addressing missing data points through imputation techniques or removal, depending on the extent of missing data.
- Outlier Detection and Treatment: Identifying and managing extreme values that could skew the results. Outliers might indicate errors or exceptional circumstances that require further investigation.
- Data Transformation: Converting data into a suitable format for analysis. This might involve standardizing units, converting date formats, and recoding categorical variables.
Data Analysis Techniques for Big Rig ROCK Report 3.12
Descriptive Statistics
Descriptive statistics provide a summary of the data using measures like:
- Mean: Average value of a dataset.
- Median: The middle value when data is ordered.
- Standard Deviation: A measure of data spread.
For example, calculating the mean fuel consumption per mile from Laser 101.7 data using descriptive statistics provides a baseline for comparison across different vehicles or drivers. Visualizations, such as histograms and box plots, help illustrate these descriptive statistics effectively.
Inferential Statistics
Inferential statistics enable us to draw conclusions about a larger population based on a sample. Techniques include:
- Hypothesis Testing: Determining if observed differences between groups are statistically significant. For instance, we could test if there's a significant difference in fuel efficiency between drivers.
- Regression Analysis: Modeling the relationship between variables to predict future outcomes. We could use regression to predict fuel consumption based on factors like speed, weight, and terrain.
These techniques reveal underlying trends and patterns often hidden in raw data, helping to identify areas for improvement.
Data Visualization and Reporting
Effective data visualization is crucial for communicating findings clearly. Suitable chart types include:
- Bar Charts: Comparing different categories (e.g., fuel consumption across different routes).
- Line Graphs: Showing trends over time (e.g., fuel efficiency over several months).
- Scatter Plots: Illustrating relationships between two variables (e.g., speed and fuel consumption).
Well-designed reports, incorporating these visualizations, simplify complex data and facilitate informed decision-making.
Interpreting Results and Drawing Conclusions from Laser 101.7 Data within Big Rig ROCK Report 3.12
Identifying Key Trends and Patterns
Analyzing Laser 101.7 data within the Big Rig ROCK Report 3.12 allows you to:
- Identify Fuel Efficiency Trends: Spot high-consumption vehicles or routes for optimization.
- Analyze Route Optimization: Identify routes with consistently higher fuel consumption or longer travel times.
- Assess Driver Performance: Pinpoint drivers with consistently poor fuel efficiency or high rates of hard braking.
By examining these trends, you can identify areas needing improvement.
Actionable Insights and Recommendations
Based on the analysis, develop actionable steps like:
- Route Optimization: Adjusting routes to avoid congested areas or inefficient paths.
- Driver Training: Implementing programs focused on fuel-efficient driving techniques.
- Preventative Maintenance: Scheduling regular maintenance to prevent costly breakdowns.
These recommendations are data-driven and focused on improving specific areas of your operation.
Limitations and Future Considerations
Acknowledge potential limitations, such as data quality issues or incomplete datasets. Suggest improvements for future analysis, including expanding data collection to incorporate additional metrics or improving data accuracy.
Conclusion: Mastering Data Analysis for Big Rig ROCK Report 3.12 and Laser 101.7 Success
Effectively analyzing Big Rig ROCK Report 3.12 data, particularly the Laser 101.7 subset, is crucial for informed decision-making in the trucking industry. By applying the data analysis and data interpretation techniques described here, you can identify key trends, improve fleet efficiency, and boost profitability. Don't let valuable data go untapped – start analyzing your Big Rig ROCK Report 3.12 and Laser 101.7 information today to unlock the full potential of your fleet. Explore advanced data analysis tools and techniques to further refine your approach. Mastering data analysis is key to mastering your fleet's success!
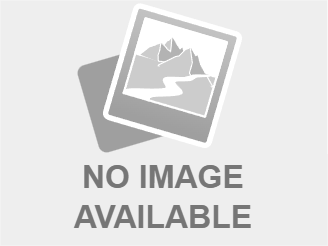
Featured Posts
-
Zak Starkey Rehired The Who Drummer Confirmed By Pete Townshend
May 23, 2025 -
2027 Tour De France Edinburghs Grand Depart
May 23, 2025 -
Everything You Need To Know About Netflixs Sirens
May 23, 2025 -
Mc Laren And Hamilton An Unresolved Issue
May 23, 2025 -
Big Rig Rock Report 3 12 99 5 The Fox
May 23, 2025
Latest Posts
-
Rb Leipzig Eyeing Erik Ten Hag Could The Former Man United Boss Take The Bundesliga Helm
May 23, 2025 -
Brian Brobbey Vs Rasmus Hojlund Ten Hags E40m Transfer Decision Scrutinized
May 23, 2025 -
Previa Instituto Lanus Analisis De La Convocatoria Y El Once Probable
May 23, 2025 -
Novedades En La Lista De Citados De Instituto Para El Partido Contra Lanus
May 23, 2025 -
Erik Ten Hags E40m Brobbey Bid A Missed Opportunity
May 23, 2025