Exploring The Cognitive Capacity Of AI: Beyond The Hype
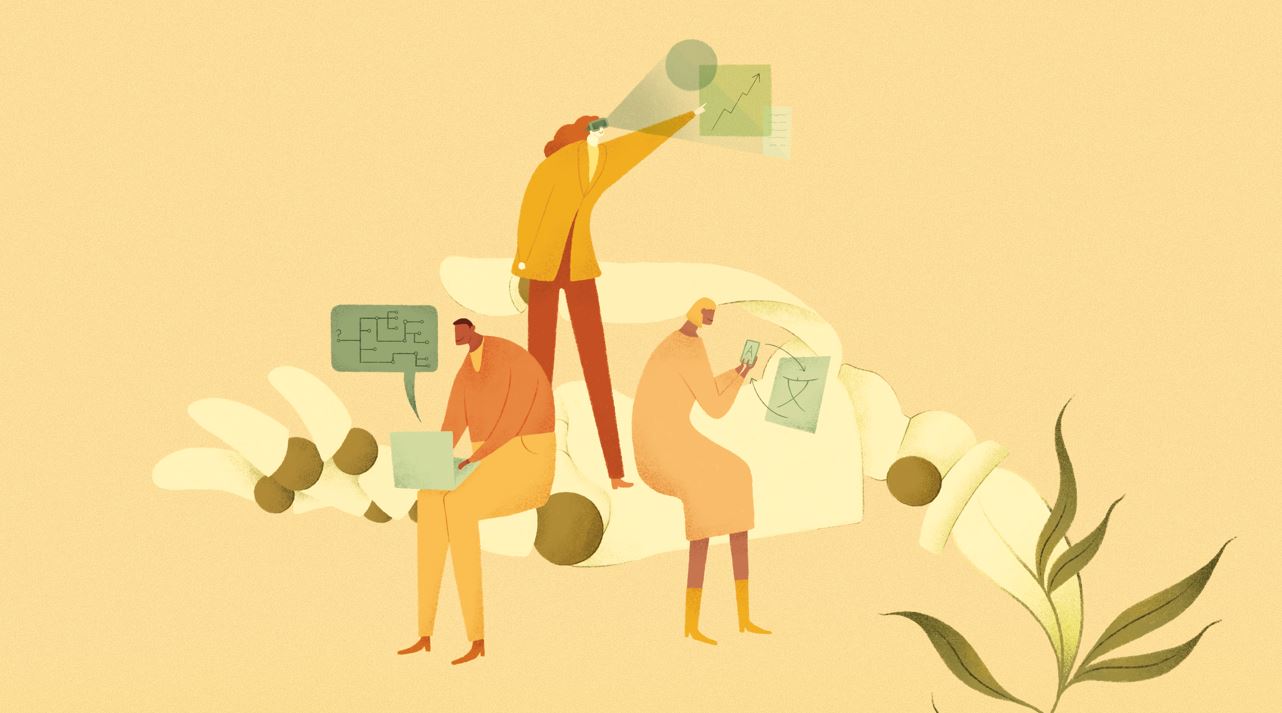
Table of Contents
Current Strengths of AI Cognitive Capabilities
H3: Machine Learning and Pattern Recognition: AI excels at identifying patterns in vast datasets, leading to breakthroughs in image recognition, natural language processing (NLP), and predictive analytics. This ability is fueled by sophisticated machine learning algorithms that can analyze massive amounts of data to identify subtle correlations and make predictions.
-
Examples of successful applications:
- Medical diagnosis: AI algorithms can analyze medical images (X-rays, MRIs) to detect diseases like cancer with remarkable accuracy.
- Fraud detection: Financial institutions use AI to identify fraudulent transactions by analyzing patterns in spending habits and account activity.
- Spam filtering: AI-powered spam filters effectively sort through emails, identifying and blocking unwanted messages with high precision.
-
Limitations: While AI excels at pattern recognition, it often struggles with nuanced understanding and context. It may misinterpret subtle cues or fail to grasp the underlying meaning behind data points, leading to errors in judgment. For example, an AI trained to identify cats in images might misclassify a picture of a lion as a cat due to shared visual features.
H3: Narrow AI vs. General AI: It's crucial to differentiate between Narrow AI (ANI) and Artificial General Intelligence (AGI). Current AI systems are primarily Narrow AI, designed to perform specific tasks exceptionally well. AGI, on the other hand, would possess human-level cognitive abilities and the capacity to learn and adapt to various tasks.
-
Examples of narrow AI:
- Self-driving cars: These systems excel at navigating roads and avoiding obstacles but lack the general intelligence to handle unexpected situations.
- Spam filters: As mentioned above, these filters are highly effective at their specific task but cannot perform other cognitive functions.
- Recommendation systems: These systems personalize content recommendations based on user preferences but don't possess broader understanding.
-
The challenge of creating AGI: Creating AGI remains a significant challenge. Current AI models lack the common sense reasoning, adaptability, and creativity of human intelligence. The development of AGI requires breakthroughs in various fields, including neuroscience, cognitive science, and computer science.
H3: Data Dependency and Bias: AI performance is heavily reliant on the quality and quantity of data used for training. However, data often contains biases that can be reflected in the resulting AI models. These biases can lead to unfair or discriminatory outcomes.
-
Examples of bias in AI algorithms:
- Facial recognition: Some facial recognition systems exhibit bias against certain racial groups, leading to inaccurate identifications.
- Loan applications: AI-powered loan applications may discriminate against certain demographics due to biases in historical loan data.
- Recruitment tools: AI-powered recruitment tools may show bias against certain genders or ethnicities if trained on biased data.
-
Methods for mitigating bias: Addressing bias requires careful curation of training data, using techniques like data augmentation and resampling to balance datasets. Furthermore, ongoing monitoring and evaluation of AI systems are crucial for identifying and correcting biases.
Limitations of Current AI Cognitive Abilities
H3: Lack of Common Sense and Reasoning: AI systems often struggle with tasks requiring basic common sense and real-world understanding. They may fail to make inferences or draw logical conclusions that are obvious to humans.
-
Examples of situations where AI fails due to lack of common sense:
- Understanding sarcasm or humor: AI may misinterpret ironic or humorous statements due to its inability to understand contextual nuances.
- Solving ambiguous problems: AI struggles with problems that require flexible reasoning and adaptation to unexpected situations.
- Making inferences based on incomplete information: AI often requires complete and structured data to make accurate predictions.
-
The complexity of integrating common sense reasoning: Integrating common sense reasoning into AI systems is a major research challenge. It requires developing AI models that can understand the world and its complexities in a way similar to humans.
H3: Emotional Intelligence and Empathy: Current AI systems lack emotional intelligence and empathy. They cannot understand or respond to human emotions effectively.
-
Challenges in developing AI with emotional intelligence: Building AI with emotional intelligence requires creating models that can recognize, interpret, and respond appropriately to human emotions. This is a complex task that requires a deeper understanding of human psychology and neuroscience.
-
The importance of emotional intelligence in human-computer interaction: Emotional intelligence is crucial for building AI systems that can interact naturally and effectively with humans. AI systems that lack emotional intelligence may appear cold, impersonal, and difficult to work with.
H3: Creativity and Innovation: AI currently demonstrates limited ability in generating truly original ideas and creative solutions. While AI can generate creative content, it typically relies on patterns and combinations learned from existing data.
-
Comparison of AI-generated content with human creativity: AI-generated art, music, and literature often lack the depth, originality, and emotional resonance of human-created works. While impressive in their technical capabilities, they often feel less "alive" and less emotionally impactful.
-
Future directions for AI research in creativity and innovation: Future research focuses on developing AI models that can go beyond pattern recognition and demonstrate genuine creativity and innovation. This involves exploring new architectures and algorithms, incorporating elements of surprise and unpredictability, and potentially integrating AI with human creative processes.
The Future of AI Cognitive Capacity
H3: Advancements in Deep Learning and Neural Networks: Ongoing advancements in deep learning and neural networks offer potential breakthroughs in AI architecture and algorithms. New approaches, such as transformers and graph neural networks, show promise in improving AI's ability to process complex information and make more nuanced decisions.
-
Examples of new approaches in deep learning:
- Transformers: These models have revolutionized NLP, enabling AI to understand and generate human language with unprecedented accuracy.
- Graph neural networks: These models are designed to process data represented as graphs, making them suitable for applications involving relationships and interactions between entities.
-
The potential impact of these advancements on AI cognition: These advancements have the potential to significantly enhance AI's cognitive abilities, enabling it to handle more complex tasks and make more sophisticated inferences.
H3: Ethical Considerations and Responsible AI Development: The development and deployment of AI raise significant ethical concerns. It's crucial to establish ethical guidelines and regulations to ensure AI is developed and used responsibly.
-
Key ethical concerns related to AI:
- Bias: As discussed earlier, AI systems can perpetuate and amplify existing societal biases.
- Privacy: AI systems often collect and process large amounts of personal data, raising concerns about privacy violations.
- Job displacement: The automation potential of AI may lead to job displacement in various sectors.
-
The role of researchers, developers, and policymakers: Researchers, developers, and policymakers must work together to establish ethical guidelines, develop robust testing methods, and create regulatory frameworks for responsible AI development and deployment.
Conclusion
This exploration of AI's cognitive capacity reveals a complex picture. While current AI systems demonstrate remarkable capabilities in specific domains, they fall short of human-level cognitive abilities in crucial areas like common sense reasoning, emotional intelligence, and creativity. Understanding these limitations is crucial for responsible AI development and deployment. Moving beyond the hype, a realistic assessment of AI's cognitive capacity paves the way for future advancements while mitigating potential risks. Continue exploring the fascinating world of AI cognitive capacity and its implications for the future. Further research into the ethical implications of AI and responsible development practices is essential for harnessing the full potential of this transformative technology.
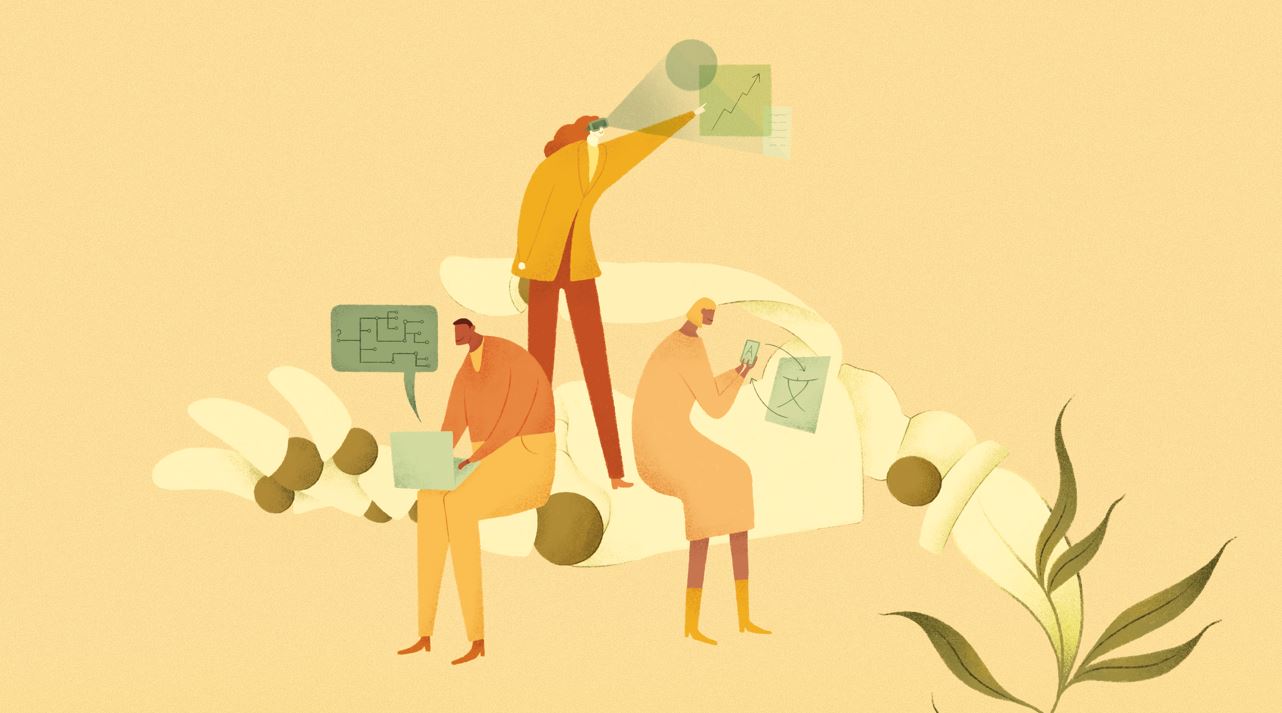
Featured Posts
-
Electric Vehicle Mandates Face Renewed Pushback From Car Dealerships
Apr 29, 2025 -
Bmw And Porsches China Challenges A Broader Look At Auto Industry Headwinds
Apr 29, 2025 -
Land Your Dream Private Credit Job 5 Essential Dos And Don Ts
Apr 29, 2025 -
Is Anthony Edwards Playing Tonight Lakers Timberwolves Injury Update
Apr 29, 2025 -
How Musks X Debt Sale Reshaped The Companys Finances
Apr 29, 2025
Latest Posts
-
New Willie Nelson Album Celebrating 92 Years With 77 Albums
Apr 29, 2025 -
Willie Nelson Pays Tribute To Longtime Roadie In Touching Documentary
Apr 29, 2025 -
Country Legend Willie Nelson Releases 77th Solo Album
Apr 29, 2025 -
New Music Willie Nelsons 77th Solo Album Out Now
Apr 29, 2025 -
New Documentary Showcases Willie Nelsons Respect For His Roadie
Apr 29, 2025