How Outdated Apps Block Your Path To Successful AI Implementation
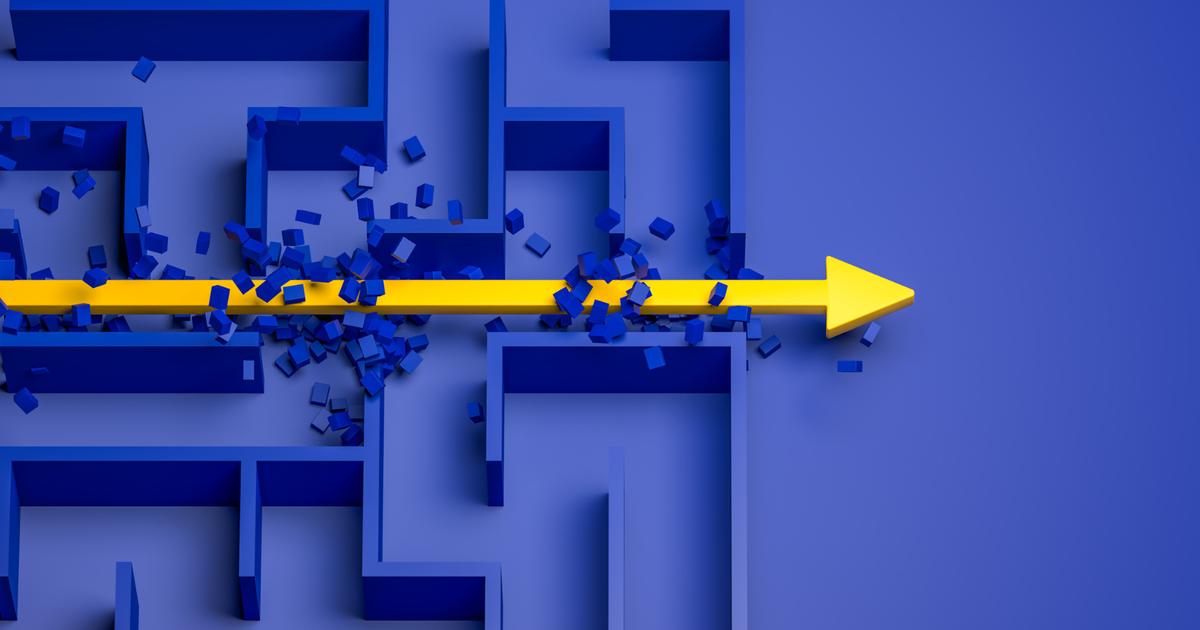
Table of Contents
Incompatibility Issues with Modern AI Tools and Infrastructure
Integrating AI solutions with outdated applications presents significant technical hurdles. Legacy systems, often built on obsolete technologies, frequently lack the necessary infrastructure to seamlessly integrate with modern AI tools and platforms. This incompatibility creates significant friction in the AI implementation process.
- Lack of APIs and integration capabilities: Many older applications lack the Application Programming Interfaces (APIs) required for smooth data exchange and communication with AI systems. This necessitates complex and costly custom integrations.
- Data format incompatibility: Outdated apps often store data in formats incompatible with modern AI algorithms, requiring extensive data migration and transformation efforts.
- Security vulnerabilities in legacy systems: Older systems often lack the robust security features needed to protect sensitive data used in AI training and deployment, increasing the risk of cybersecurity breaches.
- Scalability limitations: Legacy systems may struggle to handle the increased data volumes and processing demands associated with AI, limiting the scalability and effectiveness of AI solutions. This restricts the potential for scalable AI deployment across the entire organization.
Data Silos and the Struggle for Unified Data Access
Outdated applications frequently contribute to the creation of data silos – isolated pockets of information that are difficult to access and integrate. AI, however, thrives on comprehensive and unified data. The inability to consolidate data from disparate sources significantly impedes AI's ability to deliver accurate insights and predictions.
- Difficulty accessing and consolidating data from multiple sources: Data scattered across numerous legacy systems makes it extremely challenging to create a unified view needed for effective AI training.
- Data quality issues in legacy systems: Older systems may suffer from inconsistent data formats, missing values, and inaccuracies, negatively impacting the quality and reliability of AI models. Addressing data quality issues is crucial for robust AI implementation.
- Lack of real-time data access: Many legacy systems cannot provide the real-time data streams that many modern AI applications require for effective performance. This limits the ability to leverage real-time analytics and respond quickly to changing business needs.
- Impact on AI model accuracy and performance: The limitations in data access and quality directly affect the accuracy, reliability, and overall performance of AI models. Poor data leads to poor AI results.
Maintenance Costs and Resource Constraints
Maintaining outdated applications while simultaneously attempting AI implementation places a considerable strain on IT budgets and resources. The high costs associated with legacy system upkeep can significantly reduce the return on investment (ROI) of AI initiatives.
- High maintenance costs for legacy systems: Maintaining older systems requires significant ongoing investment in hardware, software, and specialized personnel.
- Limited developer expertise for outdated technologies: Finding developers with expertise in obsolete technologies is increasingly difficult, leading to higher labor costs and potential project delays.
- Difficulty finding compatible security patches: Older systems are often vulnerable to security threats due to a lack of available or compatible security patches. This increases the risk of data breaches and compliance violations.
- Reduced ROI on AI investments: The significant costs associated with maintaining legacy systems alongside implementing AI can drastically reduce the overall ROI of AI initiatives, undermining the business case for AI adoption. Strategic legacy system modernization is crucial to optimize the total cost of ownership (TCO).
Resistance to Change and Lack of Internal Expertise
Successfully implementing AI requires not only technological upgrades but also a significant shift in organizational culture and capabilities. Resistance to change and a lack of in-house AI expertise can present major hurdles to AI adoption.
- Employee resistance to adopting new technologies: Employees accustomed to using legacy systems may be resistant to learning and adopting new AI-driven tools and processes. Effective change management strategies are vital.
- Lack of in-house expertise in AI and modern application development: Many companies lack the necessary in-house expertise to design, implement, and maintain AI systems. Addressing the AI skills gap through training and recruitment is essential.
- Need for training and upskilling: Significant investment in employee training and upskilling programs is required to ensure that the workforce possesses the necessary skills to effectively utilize and manage AI systems.
- Importance of change management strategies: A well-defined change management strategy is crucial to address employee concerns, facilitate the adoption of new technologies, and ensure a smooth transition to AI-driven processes.
Strategies for Successful AI Implementation Despite Outdated Apps
Despite the challenges, companies can still achieve successful AI implementation even with existing outdated applications. A strategic and phased approach is key.
- Phased approach to modernization: Gradually modernize legacy systems, prioritizing those most crucial to AI integration. This reduces risk and allows for incremental improvements.
- Prioritize AI projects based on compatibility: Focus on AI initiatives that are most compatible with existing infrastructure, minimizing initial integration complexities.
- Invest in cloud migration and modernization services: Cloud migration offers the opportunity to modernize infrastructure, streamline data access, and enhance scalability for AI deployment. Leverage cloud services to modernize your systems and boost AI integration.
- Build an internal AI competency team or outsource: Develop an internal AI team with the necessary skills or outsource AI implementation to specialized AI consulting firms.
- Employ AI-powered application modernization tools: Leverage AI-powered tools to automate and accelerate the process of application modernization, improving efficiency and reducing costs.
Conclusion: Overcoming the Obstacles to Successful AI Implementation
Outdated applications present significant challenges to successful AI implementation, including incompatibility issues, data silos, high maintenance costs, and resistance to change. However, by addressing these challenges through strategic modernization efforts, companies can unlock the transformative power of AI. Assess your current application landscape, identify outdated systems, and plan for necessary modernization to achieve successful AI implementation. Consider upgrading your outdated apps to unlock the full potential of AI integration and drive digital transformation. Don’t let outdated applications block your path to successful AI implementation – take action today!
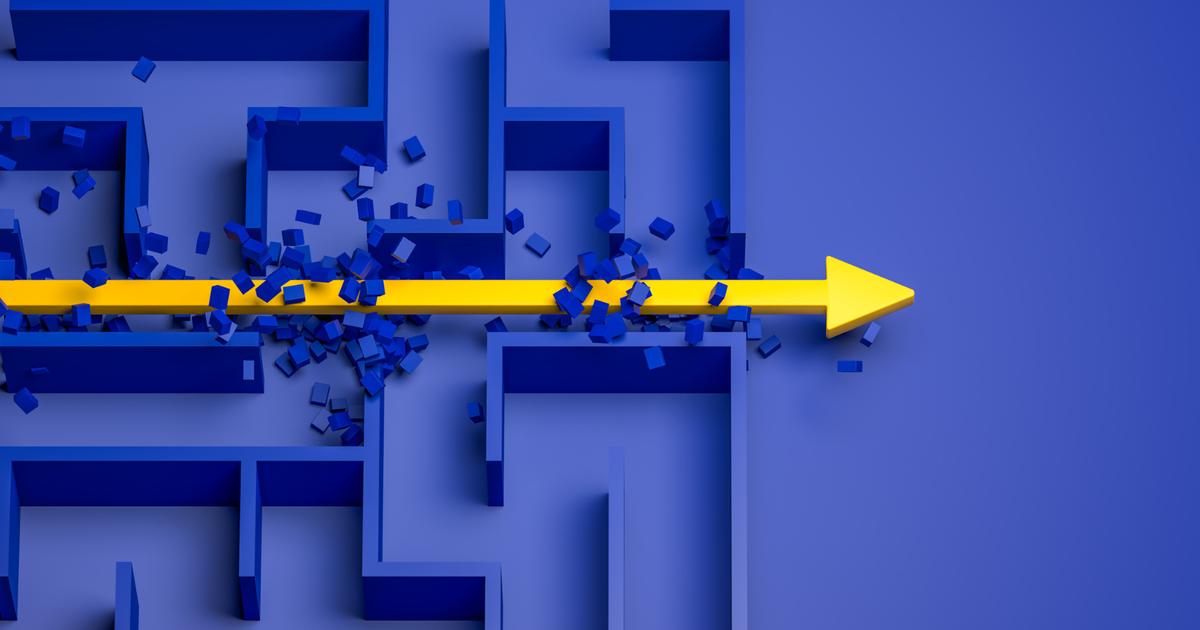
Featured Posts
-
This Shocking Food Is Worse Than Smoking Says Doctor
May 01, 2025 -
Analyzing Xrp Ripple Below 3 Investment Advice
May 01, 2025 -
Witt Jr And Garcia Lead Royals To Victory Over Guardians
May 01, 2025 -
Breda Stroomuitval 30 000 Klanten Getroffen
May 01, 2025 -
Hundreds Stranded After Kogi Train Malfunction
May 01, 2025
Latest Posts
-
Boulangerie Normande Son Poids En Chocolat Pour Le Premier Enfant De L Annee
May 02, 2025 -
Cadeau Gourmand Une Boulangerie Normande Recompense Le Premier Bebe Ne En 2024
May 02, 2025 -
Bebe Ne En Normandie Gagnez Son Poids En Chocolat
May 02, 2025 -
Cadeau Gourmand Une Boulangerie Normande Recompense Le Premier Bebe De L Annee
May 02, 2025 -
Cadeau Exceptionnel Son Poids En Chocolat Pour Le Premier Ne De L Annee Boulangerie Normande
May 02, 2025