Legacy Systems And AI: A Cloudy Outlook For Your Business
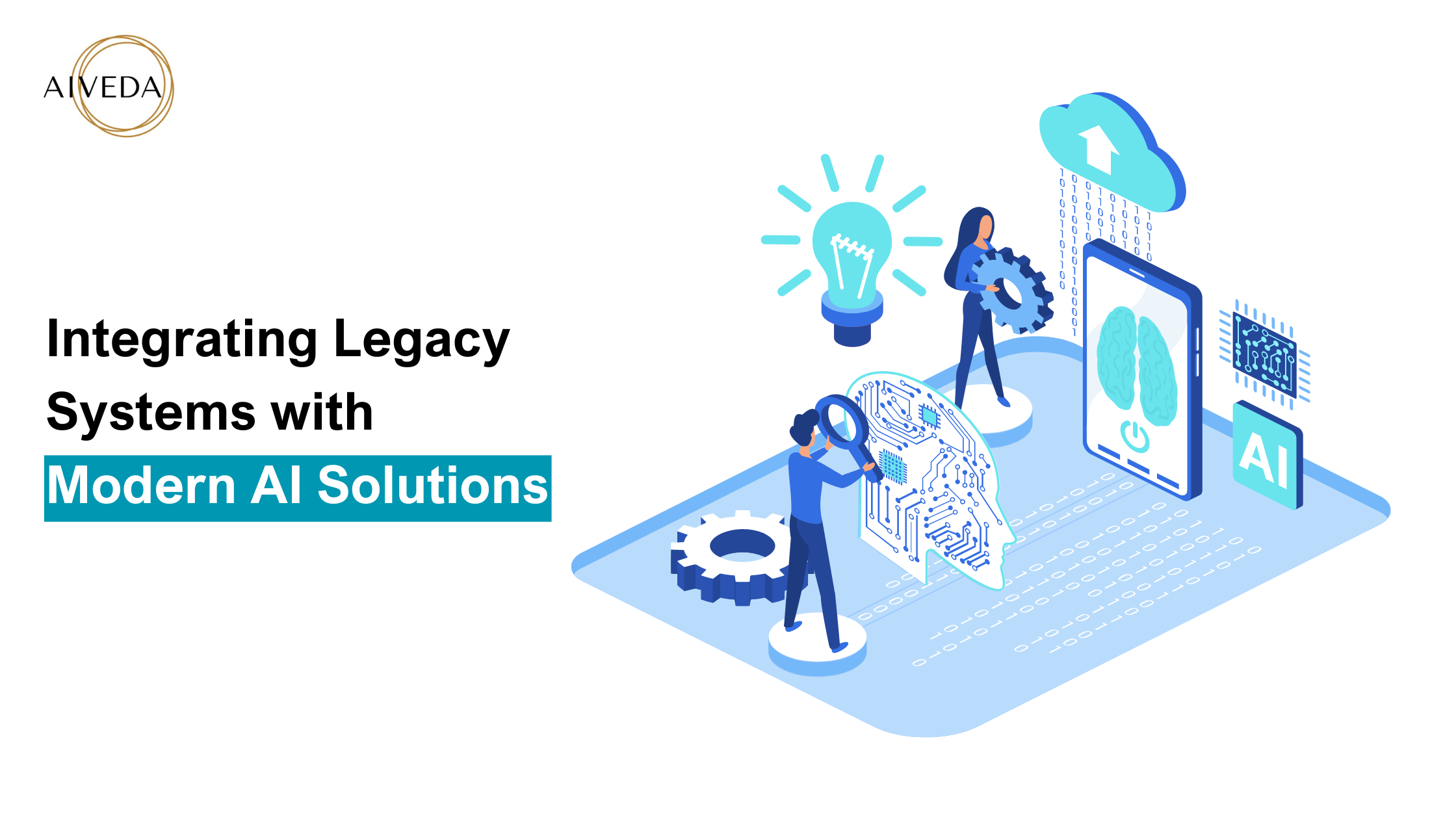
Table of Contents
Imagine this: you're a business leader striving for innovation, eager to harness the power of Artificial Intelligence (AI) to gain a competitive edge. But your path is blocked by a maze of outdated systems, legacy applications struggling to keep pace with modern demands. A recent study showed that 70% of businesses report significant challenges integrating AI due to incompatible legacy systems, increasing pressure to modernize. This highlights the critical relationship between Legacy Systems and AI, and the urgent need for strategic planning. This article argues that the incompatibility of legacy systems with AI can significantly hinder business growth and innovation, creating a cloudy outlook unless proactively addressed.
H2: The Challenges of Integrating AI with Legacy Systems
Modern businesses are under immense pressure to leverage AI for improved efficiency, predictive analytics, and automation. However, integrating AI with existing legacy systems presents numerous challenges.
H3: Data Silos and Incompatibility:
Legacy systems often trap data in isolated silos, hindering AI's ability to access and process the comprehensive information it needs for accurate analysis. This incompatibility stems from several factors:
- Incompatible Data Formats: Legacy systems might use outdated formats like flat files (.txt, .csv) or proprietary databases incompatible with modern AI tools requiring structured data (JSON, XML, Parquet).
- Lack of APIs: Many legacy applications lack Application Programming Interfaces (APIs), preventing seamless data exchange with AI platforms. This necessitates complex and costly custom integrations.
- Difficulty in Data Extraction: Extracting data from legacy systems can be time-consuming and error-prone, especially if the systems lack proper documentation or have undergone numerous modifications over time. This affects the quality of data used for AI model training.
The result? AI models trained on incomplete or fragmented data will yield inaccurate predictions and undermine the entire value proposition of AI initiatives. Imagine trying to build a robust fraud detection model with data scattered across multiple incompatible databases – the results would be unreliable at best.
H3: Scalability and Performance Issues:
Legacy systems, often built on aging hardware and software architectures, may struggle to handle the computational demands of AI workloads. This leads to:
- Slow Response Times: AI applications require fast processing speeds to deliver real-time insights. Legacy systems, with their limited processing power, can cause significant delays, hindering productivity and user experience.
- Bottlenecks: Processing large datasets needed for AI model training can overwhelm legacy systems, creating bottlenecks and reducing the efficiency of AI algorithms.
- Cost Implications: Upgrading the infrastructure to meet the demands of AI can be costly, requiring investment in new hardware, software, and potentially cloud services.
These performance limitations can significantly affect the accuracy and effectiveness of AI applications. A slow or unreliable AI system can lead to business disruptions, lost opportunities, and decreased ROI on AI investments.
H3: Security Risks and Compliance:
Integrating AI with outdated systems exacerbates existing security vulnerabilities and compliance risks:
- Data Breaches: Legacy systems often lack robust security protocols, making them susceptible to cyberattacks and data breaches. This is particularly concerning when handling sensitive customer data that fuels AI algorithms.
- Lack of Robust Security Protocols: Outdated systems may not be equipped to handle the authentication, authorization, and encryption needed to secure sensitive data in an AI environment.
- Difficulty in Maintaining Compliance: Meeting regulatory requirements like GDPR or HIPAA can be challenging when dealing with fragmented, insecure legacy systems.
Failure to address these security and compliance issues can result in significant financial losses, reputational damage, and legal repercussions.
H2: Overcoming the Hurdles: Strategies for Successful Integration
Successfully integrating AI with legacy systems requires a proactive and strategic approach.
H3: Modernization Strategies:
Several strategies can help modernize legacy systems:
- Cloud Migration: Migrating legacy applications to the cloud offers scalability, enhanced security, and access to advanced AI tools and services. However, migration can be complex and time-consuming.
- System Upgrades: Upgrading existing legacy systems with newer software and hardware can improve performance and security, but it may not address underlying architectural limitations.
- Phased Replacements: Gradually replacing legacy systems with modern, modular applications allows for a less disruptive transition, but it requires careful planning and coordination.
The optimal approach depends on factors like budget, time constraints, and the complexity of the legacy systems. Consider tools like application modernization platforms and containerization technologies for smoother transitions.
H3: Data Integration and Management:
Effective data integration is crucial for successful AI adoption. This involves:
- Data Cleansing: Cleaning and preparing data from legacy systems to ensure accuracy and consistency.
- ETL Processes: Implementing Extract, Transform, Load (ETL) processes to consolidate data from different sources into a unified format suitable for AI applications.
- Data Lakes/Data Warehouses: Utilizing data lakes or data warehouses to store and manage the consolidated data for efficient access by AI algorithms.
Tools like Informatica PowerCenter, Talend Open Studio, and Apache Kafka can assist with these data management tasks.
H3: Choosing the Right AI Solutions:
Selecting AI solutions compatible with your existing infrastructure and future modernization plans is paramount:
- Cloud-Based vs. On-Premise: Choosing between cloud-based and on-premise AI solutions depends on factors such as scalability requirements, security needs, and budget constraints.
- Integration Capabilities: Ensure that the chosen AI solution offers seamless integration with your existing systems and data sources.
- Scalability and Performance: Select AI solutions that can handle the volume and velocity of data generated by your business, while also maintaining optimal performance.
Careful consideration of these factors ensures that the chosen AI solution truly complements your modernization efforts.
3. Conclusion:
Integrating AI with legacy systems presents significant challenges, ranging from data silos and scalability issues to security risks and compliance concerns. These challenges can severely hamper business innovation and growth, resulting in a cloudy outlook for the future. However, by adopting proactive modernization strategies, implementing robust data integration processes, and selecting compatible AI solutions, businesses can overcome these hurdles and unlock the transformative potential of AI. Don't let your legacy systems cloud your future. Start planning your AI integration strategy today! Learn more about overcoming legacy system challenges and achieving successful legacy system modernization by downloading our free whitepaper: [Link to Whitepaper].
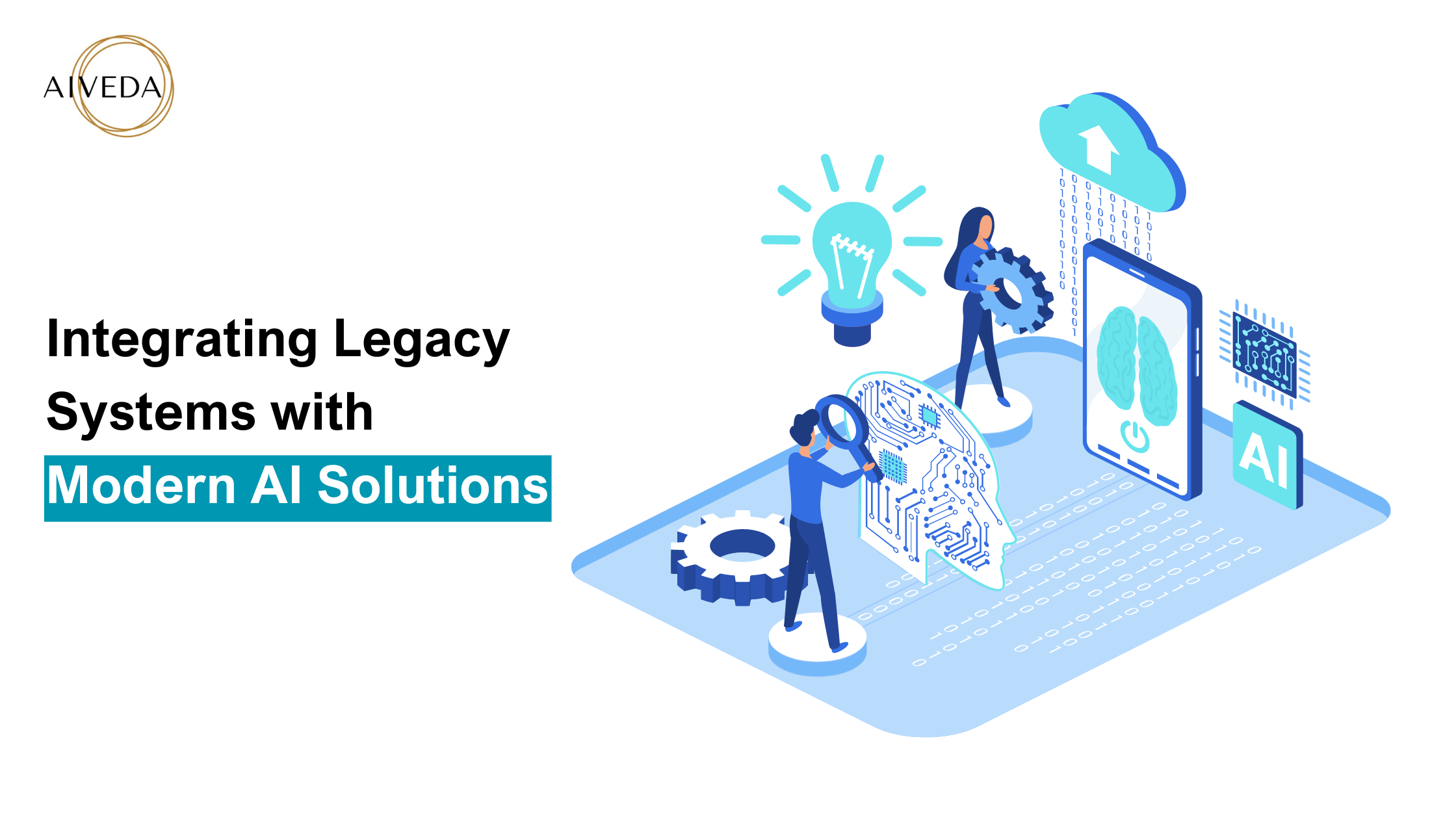
Featured Posts
-
Document Amf Mercialys 2025 E1022016 Publication Du 25 Fevrier 2025
Apr 30, 2025 -
Airbnb Domestic Bookings In Canada A 20 Increase
Apr 30, 2025 -
Zustrich Trampa Ta Zelenskogo Chomu Voni Sidili Okremo
Apr 30, 2025 -
Kamala Harris Mia After Election Democratic Insiders Express Disappointment
Apr 30, 2025 -
Swysra Artfae Qyasy Fy Asthlak Tbq Alraklyt
Apr 30, 2025
Latest Posts
-
Target Starbucks Vs Standalone 9 Key Differences
Apr 30, 2025 -
9 Ways Target Starbucks Differ From Standalone Stores
Apr 30, 2025 -
Gia Tieu Leo Thang Co Hoi Va Thach Thuc Cho Nguoi Trong Tieu
Apr 30, 2025 -
Thi Truong Tieu Hom Nay Gia Bat Tang Trien Vong Tich Cuc Cho Nong Dan
Apr 30, 2025 -
Gia Tieu Tang Niem Vui Tro Lai Voi Nong Dan
Apr 30, 2025