Turning "Poop" Into Podcast Gold: An AI-Powered Approach To Repetitive Document Analysis
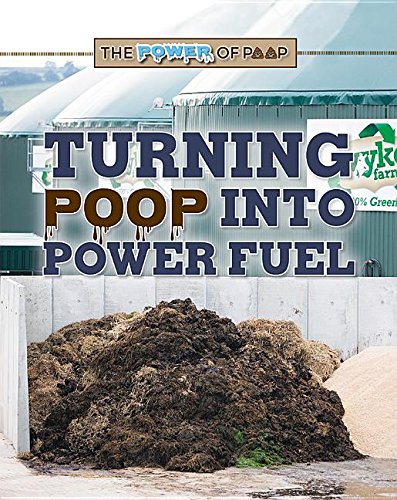
Table of Contents
The Problem with Manual Repetitive Document Analysis
Manual repetitive document analysis presents significant challenges across various aspects of the process. The sheer volume of data often leads to bottlenecks, impacting efficiency and ultimately, the bottom line.
Time Consumption and Inefficiency
Manual analysis is notoriously time-consuming, leading to significant inefficiencies. Consider the following:
- Hours spent on keyword searches: Manually searching through countless documents for specific keywords is painstaking and inefficient.
- Days spent comparing documents for inconsistencies: Identifying discrepancies between versions or across multiple documents can take days, even weeks, depending on the volume.
- Weeks spent summarizing findings: Synthesizing information from numerous documents into concise, actionable summaries requires significant time and effort.
This wasted time represents a substantial opportunity cost. Resources are diverted away from strategic activities and crucial decision-making, impacting project timelines and overall profitability.
Human Error and Inconsistency
Human error is inevitable in manual repetitive document analysis. The sheer volume of data and the monotonous nature of the work increase the likelihood of mistakes, leading to:
- Missed insights: Critical information may be overlooked due to fatigue or simple oversight.
- Incorrect conclusions: Errors in data interpretation can result in flawed conclusions and misguided decisions.
- Inconsistency across analysts and projects: Different analysts may interpret the same data differently, leading to inconsistent findings and further complicating decision-making.
These errors can have significant consequences, impacting everything from legal cases to financial forecasting.
Scalability Issues
Scaling manual repetitive document analysis to handle larger datasets is incredibly difficult. As data volumes grow, manual processes become exponentially more time-consuming and prone to errors. The limitations of manual processes become acutely apparent when dealing with:
- Increased volume of documents
- Rapidly expanding data sources
- Shorter project deadlines
This inherent lack of scalability makes manual analysis unsustainable in many modern business contexts.
AI-Powered Solutions for Efficient Document Analysis
Artificial intelligence offers a game-changing solution to the challenges of repetitive document analysis. By automating tedious tasks and providing powerful analytical capabilities, AI transforms the process from a laborious chore into a streamlined, insightful endeavor.
Natural Language Processing (NLP)
Natural Language Processing (NLP) is a crucial component of AI-powered document analysis. NLP algorithms enable computers to understand, interpret, and manipulate human language. This allows for:
- Automated information extraction: NLP can automatically extract key information, such as dates, names, locations, and other relevant entities, from unstructured text.
- Pattern identification: NLP can identify recurring themes, patterns, and sentiment within large volumes of text data.
- Automated summarization: NLP can generate concise summaries of lengthy documents, saving analysts significant time and effort.
Specific NLP tasks like sentiment analysis, entity recognition, and topic modeling further enhance the efficiency and accuracy of document analysis.
Machine Learning (ML) for Pattern Recognition
Machine learning (ML) algorithms can identify subtle patterns and anomalies within datasets that might be missed by human analysts. This capability is invaluable for:
- Anomaly detection: ML can identify unusual patterns or outliers that could indicate fraud, errors, or other significant issues.
- Predictive modeling: ML can be used to predict future trends or outcomes based on historical data, enabling proactive decision-making.
- Improved accuracy: ML algorithms learn from data, continually improving their accuracy over time.
By automating pattern recognition, ML significantly reduces the risk of human error and improves the overall accuracy of the analysis.
Automation and Workflow Optimization
AI can automate many repetitive tasks associated with document analysis, including:
- Data entry: Automatically extracting data from documents and populating databases.
- Data cleaning: Identifying and correcting inconsistencies or errors in the data.
- Data validation: Verifying the accuracy and completeness of the data.
By automating these tasks, AI frees up human analysts to focus on higher-level tasks, such as interpretation and strategic decision-making. Integrated AI-powered workflows further streamline the entire process, improving overall efficiency and collaboration. Many platforms now offer AI-driven document analysis capabilities, integrating seamlessly with existing workflows.
Practical Applications and Case Studies
The applications of AI-powered repetitive document analysis are vast and impactful across various industries.
Case Study 1: Legal Document Review
In legal settings, AI significantly streamlines contract review, discovery, and due diligence processes. AI can quickly scan hundreds of documents to identify key clauses, potential risks, and relevant precedents, saving law firms countless hours and reducing costs. One study showed a 60% reduction in review time using AI.
Case Study 2: Financial Reporting
AI automates the analysis of financial statements and reports, identifying anomalies and potential risks more efficiently than manual methods. AI can flag inconsistencies, unusual transactions, and potential fraud indicators, improving the accuracy and timeliness of financial reporting. This can lead to a 75% reduction in the time taken for financial statement analysis, according to a recent industry report.
Case Study 3: Market Research
In market research, AI helps analyze massive volumes of customer feedback, social media data, and market trends. AI can identify key themes, sentiment, and emerging patterns, providing valuable insights for product development, marketing strategies, and business decisions. Companies have reported a 40% increase in actionable insights from market research by incorporating AI.
Conclusion: Turning Data "Poop" into Podcast-Worthy Insights with AI
AI-powered solutions offer a transformative approach to repetitive document analysis, delivering significant benefits: substantial time savings, improved accuracy, increased efficiency, and superior scalability. The transformation is clear: from tedious manual tasks to insightful, data-driven decision-making. Stop wasting time on tedious tasks! Embrace AI-powered solutions to transform your repetitive document analysis and unlock valuable insights. Learn more about how AI can benefit your organization by exploring the resources available at [link to relevant resource].
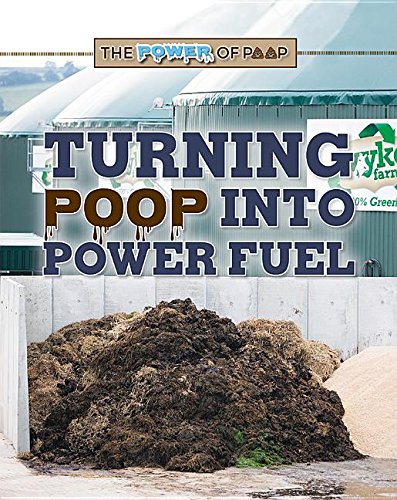
Featured Posts
-
Sluchaj Podcastu Onetu I Newsweeka Stan Wyjatkowy Dwa Razy W Tygodniu
May 07, 2025 -
Ed Shiyrn Govori Za Riana
May 07, 2025 -
My Cousin Vinny Reboot Ralph Macchio Provides Update On Potential Sequel With Joe Pesci
May 07, 2025 -
Leveraging March Madness Without Trademark Infringement
May 07, 2025 -
Rekordsmen N Kh L Po Silovym Priemam Ukhodit Iz Khokkeya
May 07, 2025
Latest Posts
-
Celebrity Special Examining The Success And Failures On Who Wants To Be A Millionaire
May 07, 2025 -
Dame Laura Kenny Reflecting On Gold Medals Mentorship And Next Steps
May 07, 2025 -
Celebrity Special On Who Wants To Be A Millionaire A Look At The Impact And Legacy
May 07, 2025 -
Who Wants To Be A Millionaire Celebrity Special How Much Do The Stars Really Win
May 07, 2025 -
Laura Kennys Olympic Success Insights From Sir Chris Hoy And Her Future Role
May 07, 2025