Understanding And Implementing The Updated CNIL Guidelines For AI Models
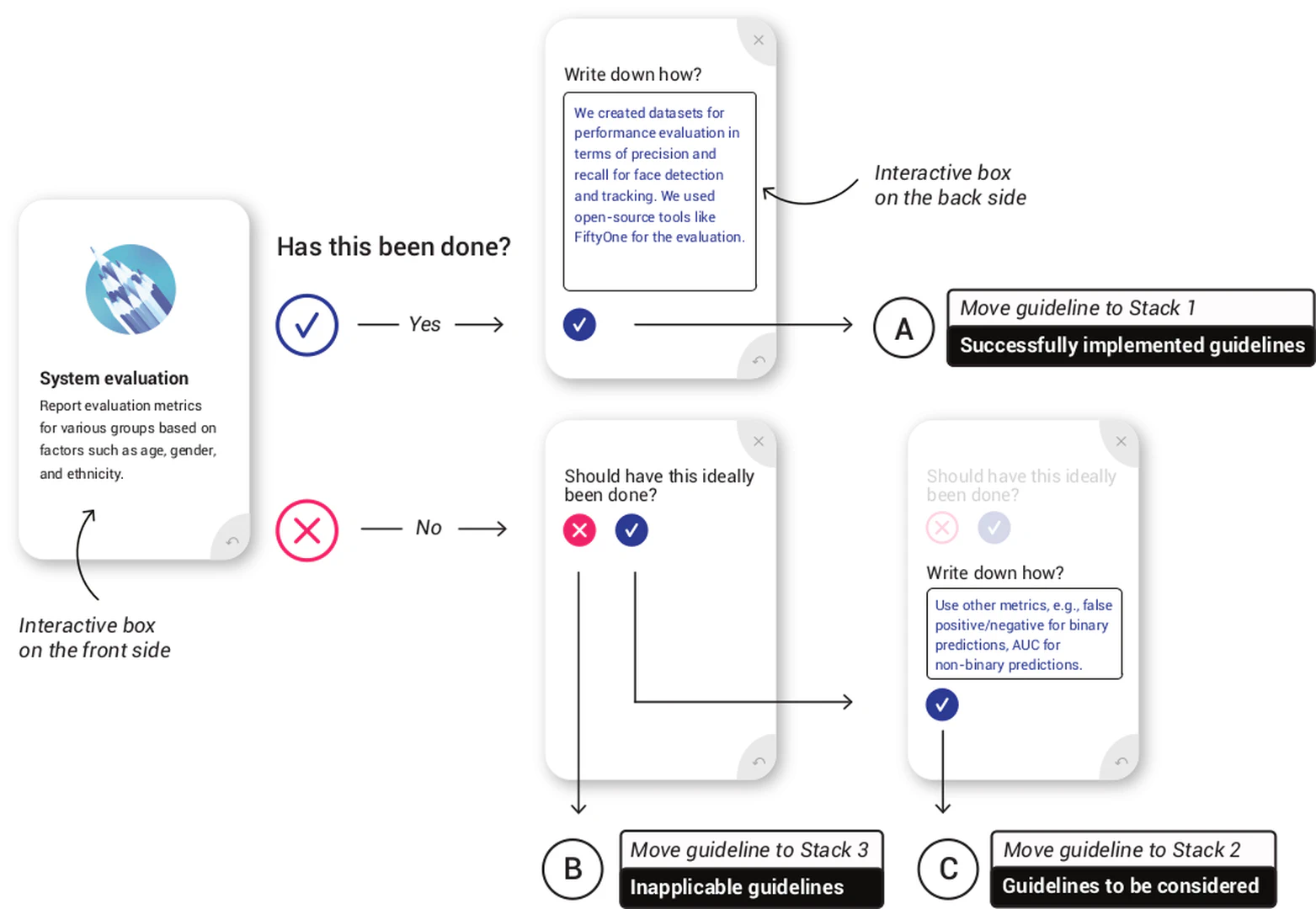
Table of Contents
Key Changes in the Updated CNIL AI Guidelines
The updated CNIL AI guidelines represent a significant evolution from previous versions, placing a stronger emphasis on transparency, data protection, and fairness. These changes reflect the increasing awareness of the potential risks associated with AI systems and the need for robust regulatory frameworks.
Increased Focus on Explainability and Transparency
The revised guidelines significantly increase the requirements for transparency and explainability in AI systems. This means providing clear and understandable information to individuals about how AI-driven decisions affecting them are made.
- Increased requirements for documenting AI model development: Meticulous record-keeping of the entire AI lifecycle, from data collection to deployment, is now mandatory.
- Emphasis on providing users with clear explanations of how AI decisions are made: Users have a right to understand the logic behind AI-driven decisions that impact them. This often involves providing simplified explanations of the process and factors considered.
- Strengthened provisions for human oversight and intervention: Human intervention mechanisms must be in place to review and potentially override AI-driven decisions, particularly in high-stakes scenarios.
Meeting these requirements necessitates detailed documentation of the AI model's architecture, the data used for training, and the decision-making process. Techniques like providing summaries of the key factors influencing a decision or using visual representations can enhance transparency. Tools like SHAP (SHapley Additive exPlanations) can help explain individual predictions.
Strengthened Data Protection Requirements
The updated CNIL AI guidelines reinforce the importance of robust data protection measures within AI systems. This aligns closely with the General Data Protection Regulation (GDPR).
- New stipulations concerning data minimization and purpose limitation: Only necessary data should be collected and used, strictly for the specified purpose.
- Enhanced safeguards for sensitive data used in AI systems: Special protection measures are required when handling sensitive data (e.g., health information, biometric data) in AI applications.
- Clearer guidance on data security and breach notification: Robust security measures are essential to prevent data breaches, and prompt notification of any breaches is mandatory.
Implementing these requirements involves rigorous data governance practices. This includes employing techniques like data anonymization and pseudonymization to protect individual identities, alongside strict access control measures and regular security audits. Compliance with GDPR's data subject rights (access, rectification, erasure) is crucial.
Emphasis on Algorithmic Bias Mitigation
The CNIL's updated guidelines emphasize the critical need to address algorithmic bias. This involves proactively identifying and mitigating potential biases in AI models to ensure fair and non-discriminatory outcomes.
- Requirements for identifying and mitigating bias in AI models: Regular assessments are required to detect potential bias in data and algorithms.
- Regular audits and assessments of AI systems for bias detection: Ongoing monitoring is crucial to identify and address emerging biases.
- Strategies for promoting fairness and non-discrimination in AI outcomes: Implementing fairness-aware algorithms and employing techniques to mitigate bias during data preprocessing and model training are critical.
Techniques for identifying bias include analyzing the data for imbalances and testing the model's performance across different demographic groups. Mitigation strategies range from data augmentation to employing fairness-aware algorithms. The CNIL expects organizations to demonstrate a commitment to ongoing bias detection and mitigation.
Practical Implementation of the CNIL AI Guidelines
Integrating the CNIL's requirements into your AI projects demands a proactive and structured approach. This involves establishing a robust compliance framework, conducting thorough training programs, and implementing ongoing monitoring.
Developing a Compliance Framework
Creating a comprehensive compliance framework is foundational to adhering to the CNIL AI Guidelines.
- Establishing internal policies and procedures for AI development and deployment: Documenting clear processes for data collection, model development, deployment, and monitoring.
- Creating a data governance structure for managing AI-related data: Designating roles and responsibilities for data management and compliance.
- Implementing data protection impact assessments (DPIAs) for high-risk AI systems: Conducting thorough assessments to identify and mitigate potential risks.
This framework should include detailed documentation of your AI systems, data flow diagrams, and risk assessments. Regularly reviewing and updating these documents is crucial for maintaining compliance.
Training and Awareness Programs
Educating your workforce about the updated guidelines is essential.
- Training employees on the updated CNIL guidelines: Providing comprehensive training to relevant personnel on the key requirements and implications of the guidelines.
- Raising awareness of ethical considerations in AI development: Promoting ethical AI development practices within the organization.
- Promoting a culture of data protection and responsible AI use within the organization: Creating an environment where data protection and ethical AI are prioritized.
Effective training programs should involve interactive sessions, case studies, and practical exercises to solidify understanding and build a strong ethical foundation.
Ongoing Monitoring and Evaluation
Continuous monitoring is key for sustained compliance.
- Regularly assessing the compliance of AI systems with the CNIL guidelines: Establishing a schedule for regular compliance checks.
- Conducting audits to identify potential vulnerabilities: Employing internal or external audits to identify areas for improvement.
- Implementing a system for continuous improvement and adaptation to evolving regulations: Staying informed about updates and adapting practices accordingly.
This ongoing process ensures that your AI systems remain compliant with the evolving CNIL AI Guidelines and best practices.
Conclusion
Understanding and effectively implementing the updated CNIL AI guidelines is crucial for responsible and ethical AI development. By adhering to these guidelines, organizations can minimize legal risks, enhance user trust, and contribute to the responsible use of AI in France and beyond. Failure to comply can lead to substantial penalties. This guide offers a foundational understanding; for detailed guidance and specific application to your AI project, consult the official CNIL documentation and seek expert advice on CNIL AI Guidelines compliance. Proactive compliance with the CNIL AI Guidelines is a strategic investment protecting your organization.
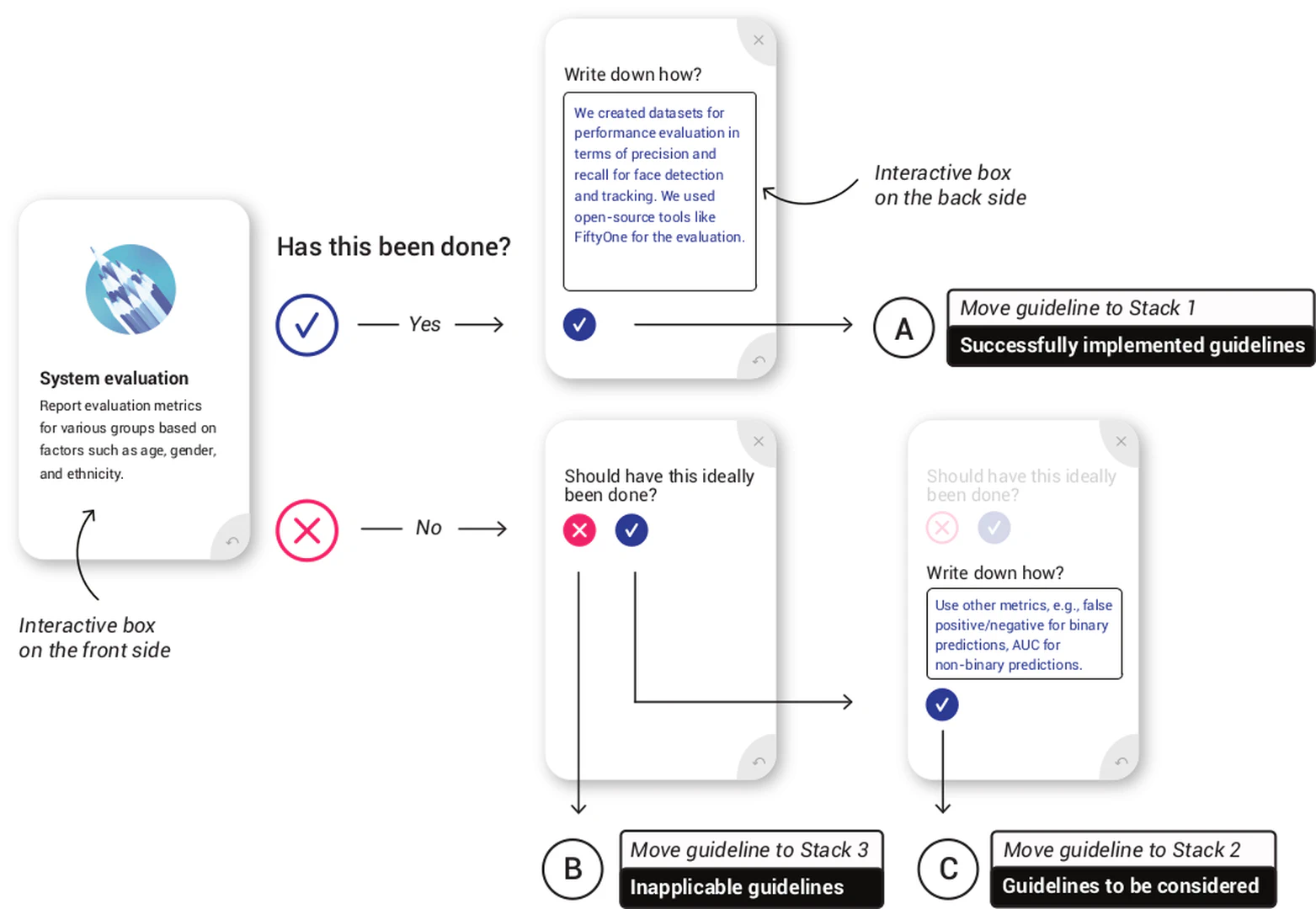
Featured Posts
-
Disney Announces Layoffs Impacting News Division And Entertainment Networks Shuttering 538
Apr 30, 2025 -
Pokhorony Papy Rimskogo Tramp Dopuskaet Vstrechu S Zelenskim
Apr 30, 2025 -
Namen Beyonce En Jay Z Verwijderd Van Diddys Aanklacht Wat Er Gebeurde
Apr 30, 2025 -
Trump Country Reels Under Federal Funding Cuts
Apr 30, 2025 -
Nevsehir De Meydana Gelen Yueksekten Duesme Kazasi Detaylar Ortaya Cikti
Apr 30, 2025
Latest Posts
-
Bof A On Stock Market Valuations A Reasoned Perspective For Investors
Apr 30, 2025 -
Stock Market Valuation Concerns Bof A Offers Reassurance
Apr 30, 2025 -
The Bof A Perspective On Elevated Stock Market Valuations
Apr 30, 2025 -
Canadian Election Results Poilievres Unexpected Defeat
Apr 30, 2025 -
Analysis Pierre Poilievres Election Loss And Its Impact
Apr 30, 2025