AI And The "Poop" Podcast: Efficiently Processing Repetitive Scatological Data
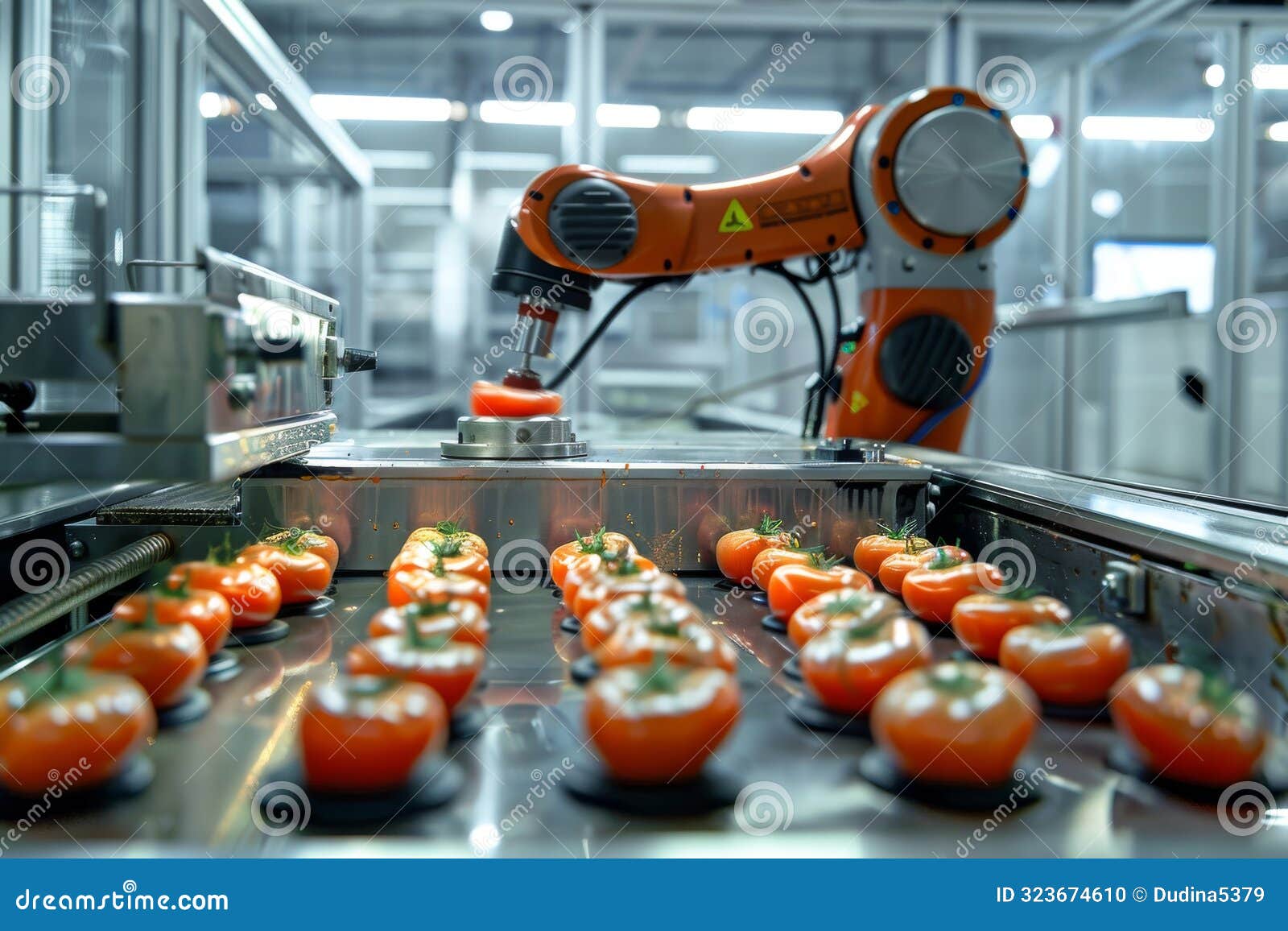
Table of Contents
Challenges of Manually Processing Scatological Data in Podcasts
Manually processing the data from podcasts focusing on scatological themes presents a significant hurdle. The sheer volume and unique nature of this data make traditional methods inefficient and prone to errors.
The Sheer Volume of Data
Manually transcribing, analyzing, and categorizing large amounts of audio data related to scatological topics is incredibly time-consuming.
- Time Constraints: Hours of audio require countless hours of manual work, hindering productivity and potentially delaying project deadlines.
- Human Error: Manual transcription and analysis inevitably lead to inconsistencies and mistakes, impacting the reliability of the final results.
- Inconsistencies in Analysis: Different researchers may interpret the same data differently, leading to a lack of standardization and comparability across studies.
Subjectivity and Interpretation
Interpreting the nuances of language and humor within scatological contexts is particularly challenging.
- Differentiating Fact from Fiction: Separating factual information about digestive health from comedic exaggeration or metaphorical language requires careful consideration and expertise.
- Contextual Understanding: Understanding the intended meaning and comedic effect of scatological jokes requires sophisticated contextual awareness, which is difficult to replicate manually on a large scale.
Identifying Patterns and Trends
Manually identifying recurring themes, trends, or patterns within a large dataset of scatological podcast data is nearly impossible.
- Topic Identification: Pinpointing the key topics discussed across numerous episodes requires extensive manual review and careful categorization.
- Sentiment Analysis: Gauging the emotional response (humor, disgust, concern) to different topics requires a nuanced understanding of language and context.
- Audience Response Tracking: Analyzing listener feedback (comments, reviews) to understand audience engagement with scatological content is extremely time-consuming.
AI Solutions for Efficient Scatological Data Processing
Fortunately, AI offers powerful tools to overcome these challenges and streamline the analysis of scatological podcast data.
Automated Transcription and Speech-to-Text
AI-powered transcription tools can significantly reduce the time and effort needed for processing audio data.
- Accuracy and Speed: Tools like Otter.ai, Descript, and Trint offer high accuracy rates and significantly faster transcription speeds compared to manual methods.
- Scalability: These tools can handle large volumes of audio data, allowing for efficient processing of entire podcast archives.
Natural Language Processing (NLP) for Sentiment Analysis
NLP techniques can analyze the emotional tone and context of scatological discussions, identifying humor, disgust, or other relevant sentiments.
- Sentiment Classification: NLP models can classify statements as positive, negative, or neutral, helping to understand the overall emotional impact of different segments of the podcast.
- Sarcasm and Irony Detection: Advanced NLP models can identify sarcasm and irony, crucial for accurate interpretation of scatological humor.
Topic Modeling and Keyword Extraction
AI can identify key themes, topics, and recurring keywords within the podcast data, providing valuable insights for content creators.
- Automated Topic Discovery: Algorithms like Latent Dirichlet Allocation (LDA) can automatically uncover underlying themes and topics within the transcribed text.
- Keyword Extraction: Techniques like TF-IDF can identify the most important keywords and phrases, revealing the most frequently discussed topics.
Data Visualization and Reporting
AI can visualize the processed data, creating clear reports and insights.
- Interactive Dashboards: Data visualization tools can create interactive dashboards showcasing key findings, allowing for easy exploration and analysis.
- Customizable Reports: Reports can be tailored to specific needs, focusing on particular aspects of the data (e.g., sentiment trends over time, frequency of specific keywords).
Conclusion: Leveraging AI for Superior Scatological Data Analysis
Manually processing scatological podcast data is time-consuming, error-prone, and subjective. AI offers efficient solutions, dramatically improving accuracy and revealing deeper insights. Through automated transcription, NLP-powered sentiment analysis, topic modeling, and data visualization, AI unlocks the potential hidden within this unique dataset. The benefits include significant time savings, increased accuracy, and the ability to identify meaningful patterns and trends that would be impossible to detect manually. Unlock the potential of your scatological data with AI – start exploring efficient processing solutions today!
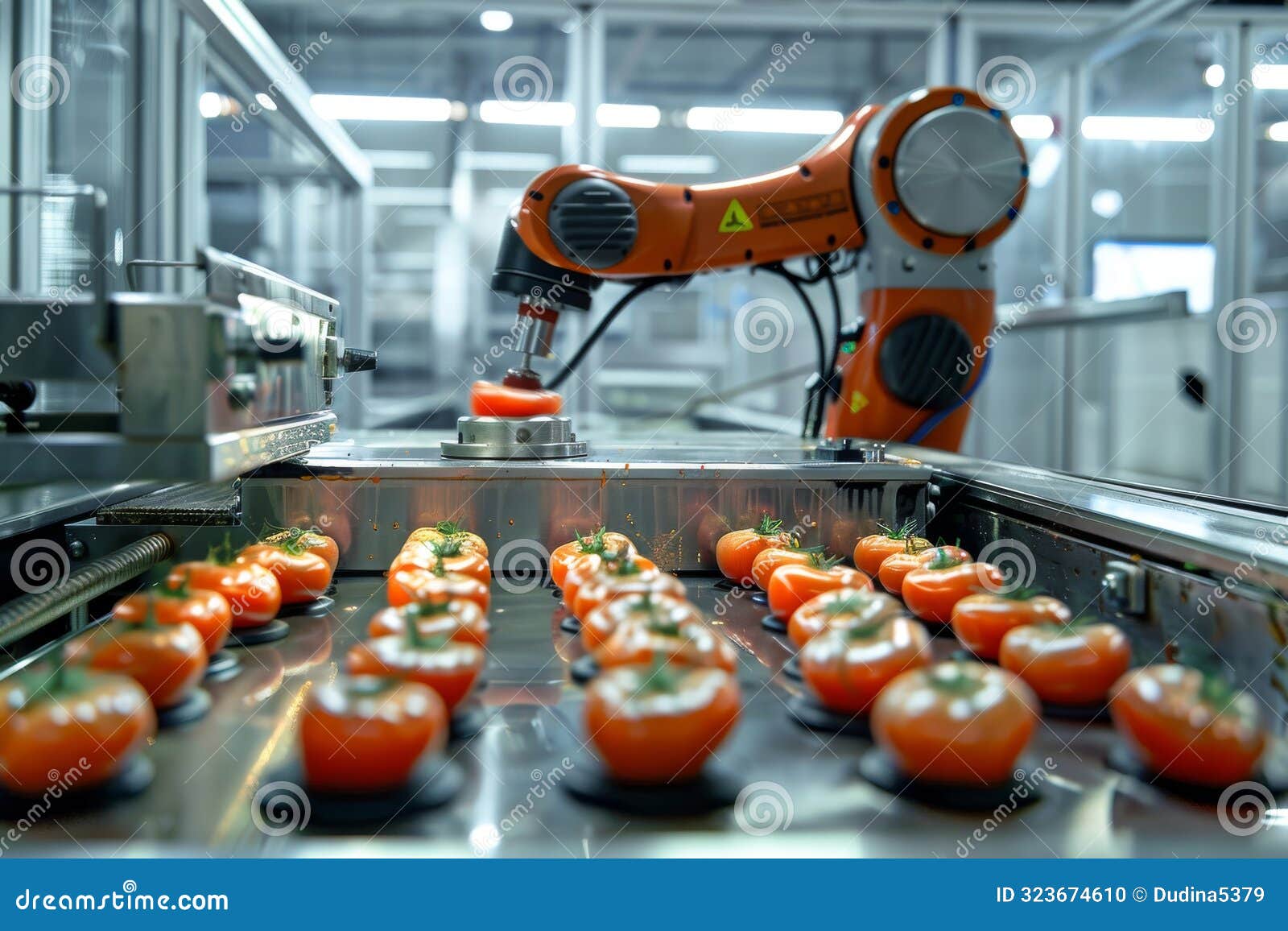
Featured Posts
-
Chivas Regals New Brand Ambassador Formula 1 Star Charles Leclerc
May 20, 2025 -
Agatha Christies Poirot Adaptations Books And Characters
May 20, 2025 -
Ryanair Tariff War Biggest Threat Stock Buyback Planned
May 20, 2025 -
6 Billion Investment Public Works Ministry Awards Sea And River Defence Contracts
May 20, 2025 -
Biarritz Trois Journees D Echanges Pour Les Femmes Autour Du 8 Mars
May 20, 2025
Latest Posts
-
The Impact Of Layoffs On An Abc News Show
May 20, 2025 -
Impact Of Mass Layoffs On Abc News Shows Programming
May 20, 2025 -
Mass Layoffs Cast Shadow On Future Of Abc News Program
May 20, 2025 -
Abc News Show Future In Jeopardy After Staff Cuts
May 20, 2025 -
Abc News Shows Future In Jeopardy After Staff Cuts
May 20, 2025