AI's Limited Thinking: A Revealing Look At Artificial Intelligence
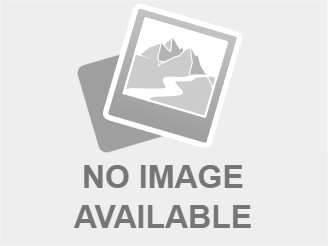
Table of Contents
Lack of Common Sense Reasoning
One of the most significant limitations of AI is its striking lack of common sense reasoning. While AI excels at specific tasks, it struggles with tasks that humans find intuitively simple. This gap highlights the significant difference between the capabilities of current AI systems and true human intelligence. AI's limited reasoning capabilities are a major hurdle in achieving artificial general intelligence (AGI).
- AI struggles with tasks that humans find intuitively simple: Consider asking an AI to understand a joke, recognize sarcasm, or interpret a complex social situation. These nuanced tasks require a level of common sense and contextual understanding that current AI systems largely lack.
- Narrow vs. General AI: Most existing AI is "narrow" AI, designed for specific tasks. General AI, on the other hand, would possess human-level intelligence and common sense reasoning – a goal that remains elusive. The limitations of narrow AI are evident in its inability to generalize knowledge and apply it to new, unseen situations.
- Examples of AI failures due to lack of common sense: Numerous instances demonstrate AI's struggles with common sense. For example, an AI might fail to understand that a statement like "the cat sat on the mat" implies the cat is likely a living creature, not a fictional character. Such failures underscore AI's limited reasoning capabilities.
The Problem of Contextual Understanding
Another critical aspect of AI's limited thinking is its difficulty in understanding nuanced language and context. While natural language processing (NLP) has made significant strides, it still struggles with the complexities of human communication. AI's understanding of context remains a significant challenge.
- Challenges of NLP and its limitations: NLP aims to enable computers to understand, interpret, and generate human language. However, NLP struggles with subtleties like idioms, metaphors, and sarcasm, which rely heavily on context and implicit meaning. AI's contextual limitations hinder its ability to accurately interpret human communication.
- Examples of AI misinterpreting subtle cues: A simple sentence like "I'm feeling a bit under the weather" might be misinterpreted by an AI that lacks contextual understanding, failing to recognize the implied meaning of illness. This highlights the challenges of semantic ambiguity for AI systems.
- The role of emotional intelligence: Human communication is often infused with emotion. AI, lacking emotional intelligence, struggles to interpret the emotional undertones in language and accurately gauge the speaker's intent. This absence of emotional understanding contributes significantly to AI's contextual limitations.
Dependence on Data and Algorithms
The performance of AI systems is heavily reliant on the data they are trained on and the algorithms used to process that data. This dependence introduces several limitations. AI's dependence on data is a double-edged sword.
- Bias in AI algorithms and datasets: If the data used to train an AI is biased, the AI will inevitably inherit and amplify those biases, leading to unfair or inaccurate outcomes. This is a significant concern in areas like criminal justice, loan applications, and hiring processes.
- How biased data leads to inaccurate or unfair outcomes: Biased data can lead to AI systems making discriminatory decisions, perpetuating existing social inequalities. For instance, if a facial recognition system is trained primarily on images of one demographic group, it may perform poorly on other groups, leading to misidentification and unfair treatment.
- Limitations of current machine learning techniques: Current machine learning techniques often struggle with handling noisy or incomplete data, leading to inaccuracies and unpredictable outcomes. Furthermore, the "black box" nature of some algorithms makes it difficult to understand why an AI makes a particular decision, hindering accountability and transparency.
Inability to Learn and Adapt Independently
A key limitation of current AI systems is their inability to learn and adapt independently in the same way humans do. While AI can learn from data, this learning is often heavily supervised. AI's learning limitations are crucial to consider.
- Supervised vs. unsupervised learning: Supervised learning involves training an AI on a labeled dataset, where the desired outcomes are explicitly provided. Unsupervised learning, on the other hand, involves training an AI on unlabeled data, allowing it to discover patterns and relationships on its own. Current AI systems predominantly rely on supervised learning, limiting their ability to adapt to new situations without explicit guidance.
- Challenges of truly independent learning and adaptation: Achieving true independent learning and adaptation remains a major challenge in AI research. Creating AI systems that can learn and adapt without constant human intervention requires breakthroughs in areas such as reinforcement learning and transfer learning.
- Ongoing research into more autonomous learning systems: Research is ongoing to develop more autonomous learning systems capable of learning and adapting independently. This involves developing algorithms that can handle uncertainty, learn from limited data, and generalize knowledge to new contexts.
Conclusion
Understanding AI's limited thinking—its lack of common sense reasoning, its difficulties with contextual understanding, its dependence on data and algorithms, and its inability to learn and adapt independently—is crucial for responsible development and deployment. AI’s cognitive constraints are significant. While AI has shown remarkable progress, it's vital to acknowledge its limitations. Continue exploring the complexities of artificial intelligence and join the conversation about the future of AI's capabilities. Learn more about the ongoing research into overcoming AI's limited thinking and the pursuit of truly intelligent systems. The future of AI thinking depends on acknowledging and addressing these limitations.
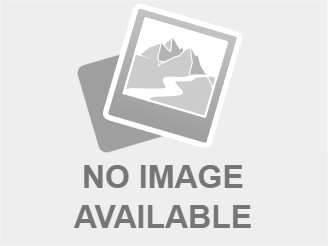
Featured Posts
-
Silver Lake Investment Fuels Khaznas Saudi Data Center Expansion
Apr 29, 2025 -
Negeri Sembilan The Next Big Data Center Location In Malaysia
Apr 29, 2025 -
Vehicle Problem Delays Blue Origins Upcoming Rocket Launch
Apr 29, 2025 -
Understanding The Treasury Market After April 8th
Apr 29, 2025 -
Akesos Disappointing Clinical Trial Results Lead To Stock Plunge
Apr 29, 2025
Latest Posts
-
Nyt Strands Puzzle Hints And Answers For March 3 2025
Apr 29, 2025 -
Solve Nyt Strands Hints And Answers For March 3rd 2025
Apr 29, 2025 -
Nyt Strands March 3 2025 Complete Answers And Hints
Apr 29, 2025 -
Nyt Spelling Bee Spangram March 13 2025 Find The Perfect Word
Apr 29, 2025 -
Nyt Strands Solutions Hints And Answers For March 3 2025
Apr 29, 2025