The Illusion Of Intelligence: How AI Works And Its Cognitive Limitations
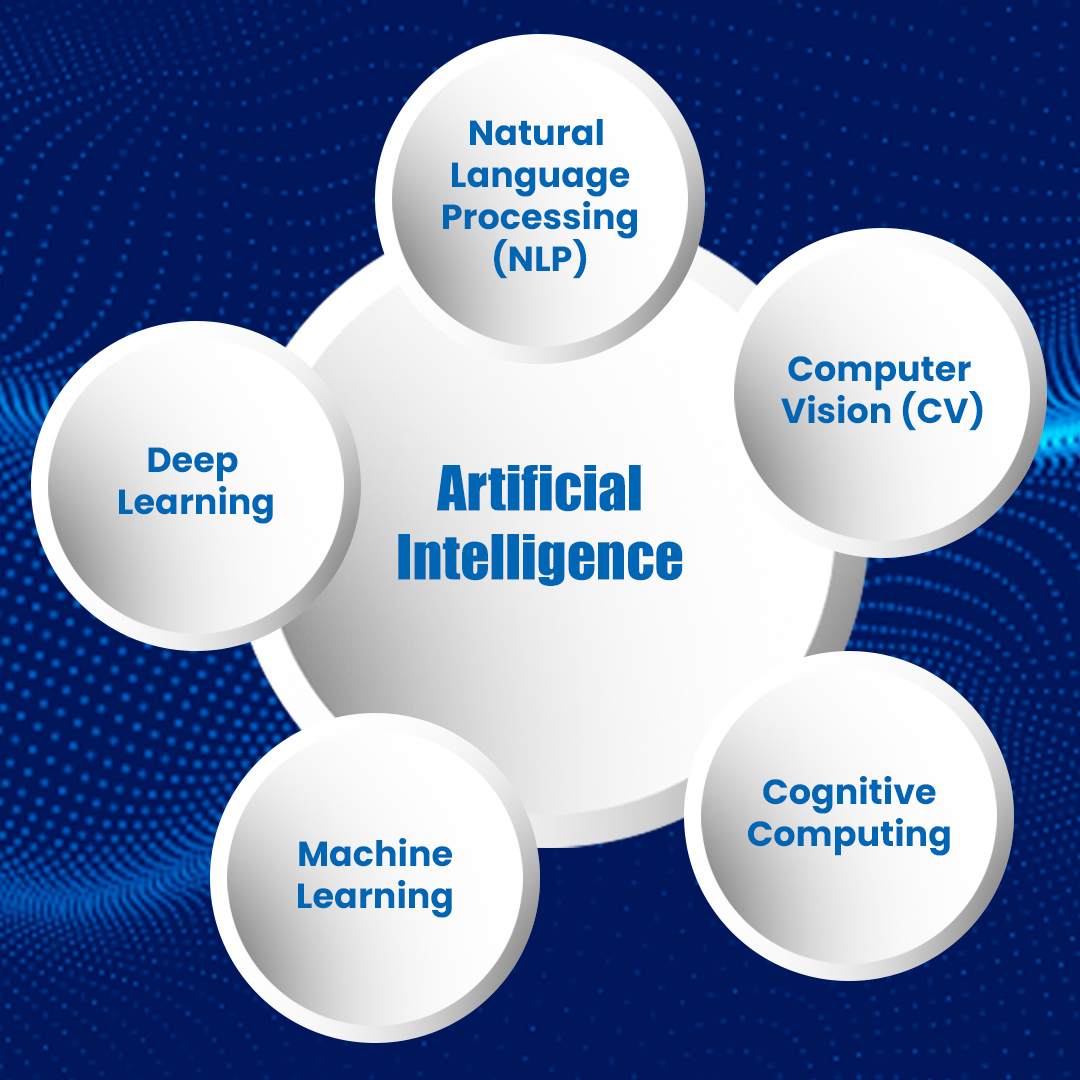
Table of Contents
How AI "Learns": The Mechanics of Machine Learning
At the heart of AI's capabilities lies machine learning (ML), a field dedicated to enabling computers to learn from data without explicit programming. Several key approaches drive ML:
-
Supervised Learning: This involves training an algorithm on a labeled dataset – data where inputs are paired with their corresponding outputs. The algorithm learns to map inputs to outputs, predicting the outcome for new, unseen data. For example, a spam filter is trained on emails labeled as "spam" or "not spam," learning to classify new emails accordingly.
-
Unsupervised Learning: This approach deals with unlabeled data, allowing the algorithm to discover patterns and structures without explicit guidance. Clustering algorithms, for example, group similar data points together, revealing hidden relationships. Recommendation systems often leverage unsupervised learning to identify user preferences based on their past behavior.
-
Reinforcement Learning: This involves an agent learning to interact with an environment to maximize a reward signal. The agent learns through trial and error, adjusting its actions based on the feedback it receives. Games like chess and Go have seen significant advancements thanks to reinforcement learning algorithms.
Machine learning algorithms, such as deep learning using neural networks, are crucial components powering these learning methods. Deep learning, in particular, utilizes networks with many layers to analyze complex data, enabling sophisticated applications:
- Image recognition: Convolutional neural networks excel at identifying objects and features in images, powering applications like self-driving cars and medical image analysis.
- Natural Language Processing (NLP): Recurrent neural networks are used for tasks like machine translation, text summarization, and chatbot development.
- Recommendation systems: Collaborative filtering, a type of unsupervised learning, recommends products or services based on user preferences and similarities with other users.
The success of these AI models heavily relies on big data analytics. Massive data sets and training data are essential to train effective algorithms.
The Limitations of Current AI: Where AI Falls Short
Despite remarkable advancements, current AI systems suffer from significant limitations. They often lack crucial aspects of human intelligence:
-
Cognitive Limitations: AI struggles with common sense and reasoning. While it can excel at specific tasks, it lacks the broad understanding and general knowledge necessary to navigate complex situations.
-
Contextual Understanding: AI systems often falter when confronted with ambiguity or nuances requiring contextual understanding. They may misinterpret subtle cues or fail to account for the overall context of a situation.
-
Real-World Applications: The limitations become strikingly apparent in real-world scenarios requiring adaptability and general intelligence. For instance, an AI-powered self-driving car might struggle to navigate an unexpected obstacle or understand the subtle cues of a pedestrian's intentions.
Examples of AI failures highlight these deficiencies:
- Bias in algorithms: AI systems trained on biased data can perpetuate and amplify existing societal biases, leading to unfair or discriminatory outcomes.
- Inability to handle unexpected situations: AI systems often fail to cope with situations that deviate from their training data, resulting in unpredictable behavior.
- Misinterpretations of ambiguous language: The nuanced nature of human language often proves challenging for AI systems, leading to misunderstandings and errors.
The "black box" problem further complicates matters. The complexity of some machine learning algorithms makes it difficult to understand their decision-making processes, hindering explainable AI, transparency, and effective bias detection.
The Difference Between AI and Human Intelligence
The gap between artificial and human intelligence remains vast. While AI excels at specific tasks, it falls short in areas requiring:
- Creativity: AI can generate novel outputs, but it lacks the genuine creativity and innovative thinking of the human mind.
- Emotional Intelligence: AI systems lack emotional understanding and empathy, hindering their ability to navigate social interactions effectively.
- Consciousness: Current AI systems lack self-awareness and consciousness, fundamental aspects of human intelligence.
- Adaptability: While AI can adapt to some extent, it lacks the flexibility and general adaptability of the human mind.
Artificial general intelligence (AGI), a hypothetical AI possessing human-level intelligence, remains a distant goal. Current AI lacks general intelligence, focusing instead on narrow, specialized tasks. The limitations are particularly pronounced in areas requiring genuine understanding, empathy, and moral judgment:
- Ethics: AI systems raise important ethical questions regarding bias, accountability, and the potential for misuse.
- Morality: Current AI lacks the capacity for moral reasoning and judgment, crucial for navigating complex ethical dilemmas.
- Emotional Intelligence: The absence of emotional intelligence limits AI's ability to understand and respond appropriately to human emotions and needs.
Examples illustrating the contrasting capabilities of AI and humans include:
- Problem-solving: Humans excel at solving novel problems requiring creativity, insight, and common sense, while AI typically relies on pre-programmed solutions or pattern recognition.
- Decision-making under uncertainty: Humans use intuition, experience, and emotional intelligence to navigate uncertainty, while AI often struggles with ambiguous or incomplete information.
- Social interaction: Humans demonstrate sophisticated social skills, emotional intelligence, and empathy, enabling complex interactions, whereas AI's social interaction abilities remain limited.
Beyond the Illusion: Understanding the True Potential of AI
In conclusion, AI's impressive capabilities often mask its fundamental reliance on algorithms and data. While AI excels at specific tasks, its current cognitive limitations highlight the vast difference between artificial and human intelligence. Understanding both the capabilities and limitations of AI is crucial to avoid unrealistic expectations and ensure responsible development. The "illusion of intelligence" must be dispelled to pave the way for the ethical and beneficial application of this powerful technology.
To continue exploring the fascinating world of AI and its implications, we encourage you to delve into further resources on AI ethics and the future of AI. Let's move beyond the illusion and embrace a future where AI's true potential is harnessed responsibly. [Link to relevant resources on AI ethics and limitations]
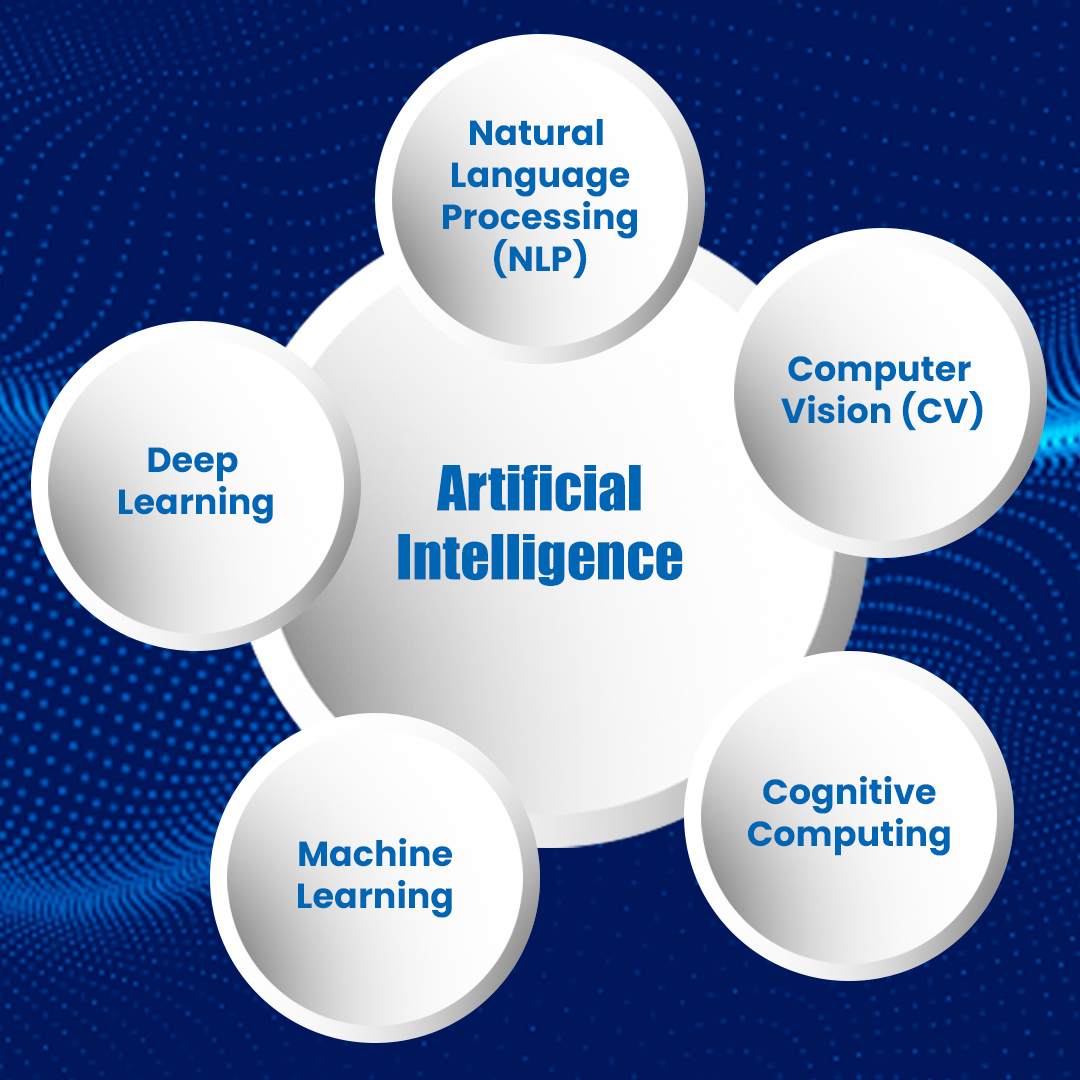
Featured Posts
-
Kuxius Solid State Power Bank Performance Price And Longevity Compared
Apr 29, 2025 -
High Profile Office365 Hack Millions Stolen Investigation Underway
Apr 29, 2025 -
Khazna Data Centers Saudi Arabia Expansion Plans After Silver Lake Deal
Apr 29, 2025 -
The Role Of Tax Credits In Attracting Film And Tv Production To Minnesota
Apr 29, 2025 -
Chinas Nuclear Energy Sector Expands With Approval Of 10 New Reactors
Apr 29, 2025
Latest Posts
-
New Willie Nelson Album Celebrating 92 Years With 77 Albums
Apr 29, 2025 -
Willie Nelson Pays Tribute To Longtime Roadie In Touching Documentary
Apr 29, 2025 -
Country Legend Willie Nelson Releases 77th Solo Album
Apr 29, 2025 -
New Music Willie Nelsons 77th Solo Album Out Now
Apr 29, 2025 -
New Documentary Showcases Willie Nelsons Respect For His Roadie
Apr 29, 2025