The Reality Of AI Cognition: What We Know And What We Don't
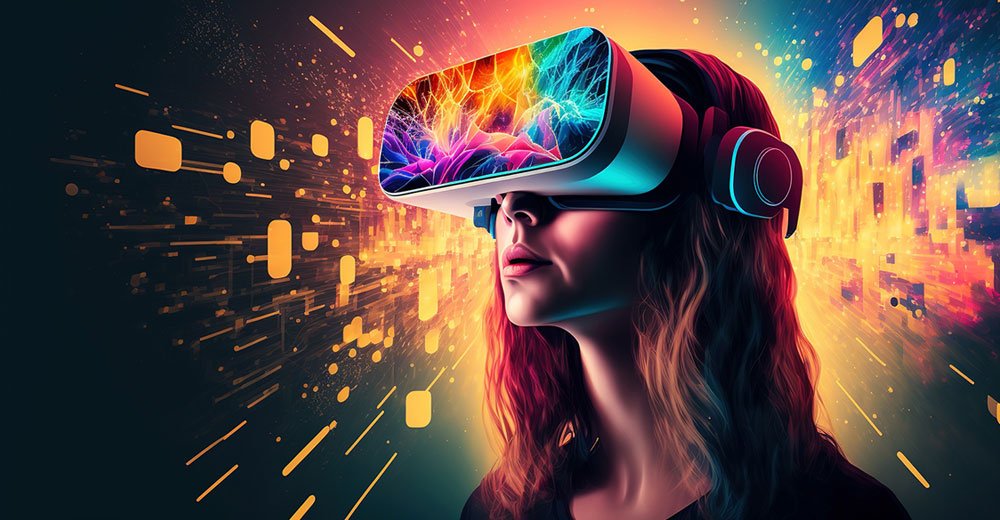
Table of Contents
What We Know About AI Cognition:
Machine Learning and its Cognitive Capabilities:
Machine learning (ML) is the cornerstone of many AI systems. ML algorithms allow AI to learn from data without explicit programming. Instead of relying on pre-defined rules, these systems identify patterns, make predictions, and improve their performance over time. This learning process mirrors, to a degree, human cognitive development.
- Supervised learning: The algorithm learns from labeled data, where inputs are paired with desired outputs. Examples include image recognition (identifying cats vs. dogs) and spam filtering.
- Unsupervised learning: The algorithm finds patterns in unlabeled data, such as clustering similar customers for targeted marketing.
- Reinforcement learning: The algorithm learns through trial and error, receiving rewards for desirable actions and penalties for undesirable ones. This is used extensively in robotics and game playing (e.g., AlphaGo).
These diverse AI algorithms, powered by cognitive computing and often implemented using artificial neural networks, are revolutionizing various sectors. The advancements in deep learning build upon this foundation.
Deep Learning and the Quest for Artificial General Intelligence (AGI):
Deep learning, a subset of machine learning, utilizes artificial neural networks with multiple layers to analyze complex data. This ability to process vast amounts of information allows deep learning models to achieve impressive results in areas like image recognition, natural language processing (NLP), and game playing. The potential of deep learning to achieve Artificial General Intelligence (AGI) – an AI with human-level cognitive abilities – is a major focus of current research.
- Convolutional Neural Networks (CNNs): Excellent at image and video analysis.
- Recurrent Neural Networks (RNNs): Specialized for sequential data like text and speech.
While systems like AlphaGo demonstrate remarkable capabilities in specific domains, the path to AGI remains challenging. Neural networks, although powerful, still lack the generalizability and adaptability of human cognition.
Current Achievements in AI Cognition:
AI systems are already showcasing impressive cognitive skills:
- Image recognition and object detection: AI can identify objects and faces in images with remarkable accuracy, fueling applications in self-driving cars and medical imaging.
- Speech recognition and natural language understanding: AI-powered virtual assistants and language translation tools demonstrate impressive progress in understanding and generating human language.
- Game playing and strategic decision-making: AI has surpassed human champions in games like chess and Go, highlighting its ability to learn complex strategies.
- Robotics and automated tasks: AI-powered robots are increasingly capable of performing complex tasks in various industries, from manufacturing to surgery. These AI applications represent a significant leap forward in computer vision, speech recognition, and natural language understanding.
What We Don't Know About AI Cognition:
The Limitations of Current AI Systems:
Despite significant progress, current AI systems have fundamental limitations:
- Common sense reasoning and real-world understanding: AI struggles with tasks that humans find trivial, such as understanding context, making inferences, and dealing with ambiguity. This is a key difference between Artificial Narrow Intelligence (ANI), which excels in specific tasks, and the sought-after AGI.
- Emotional intelligence and social interaction: AI lacks the capacity for empathy, emotional understanding, and nuanced social interaction.
- Creativity, imagination, and abstract thought: AI excels at pattern recognition, but struggles with tasks requiring creativity, original thinking, and abstract reasoning.
- Generalization and adaptation to novel situations: AI models often perform poorly when confronted with situations that differ significantly from their training data.
The Ethical Considerations of Advanced AI Cognition:
The rapid advancement of AI cognition raises crucial ethical questions:
- Bias and fairness in algorithms: AI systems can inherit and amplify biases present in their training data, leading to unfair or discriminatory outcomes. Addressing algorithmic bias is paramount.
- Job displacement and economic impact: Automation driven by AI could lead to significant job losses in various sectors, requiring proactive strategies for workforce adaptation.
- Privacy concerns and data security: The use of AI systems often involves the collection and analysis of vast amounts of personal data, raising concerns about privacy and security.
- Autonomous weapons systems and their potential consequences: The development of lethal autonomous weapons raises serious ethical and security concerns. AI safety and responsible AI ethics are crucial considerations.
The Future of AI Cognition: Open Questions and Research Directions:
Overcoming the limitations of current AI systems requires ongoing research:
- Neuro-symbolic AI: Integrating the strengths of neural networks and symbolic reasoning to achieve more robust and explainable AI systems.
- Explainable AI (XAI): Developing methods to make the decision-making processes of AI systems more transparent and understandable.
- Quantum computing for AI: Exploring the potential of quantum computing to enhance the capabilities of AI algorithms and solve complex problems currently intractable for classical computers.
These are just some of the many exciting avenues of AI research shaping the future of AI.
Conclusion: Understanding the Landscape of AI Cognition
The field of AI cognition is marked by both incredible progress and significant challenges. While AI systems have demonstrated impressive cognitive abilities in specific domains, achieving true human-level intelligence remains a distant goal. Addressing the ethical implications of advanced AI systems is crucial to ensure that this transformative technology benefits all of humanity. Continue your exploration of AI cognition by researching the latest breakthroughs and engaging in discussions about the future of this rapidly evolving field. Understanding the complexities of AI cognition is vital for navigating the opportunities and challenges it presents.
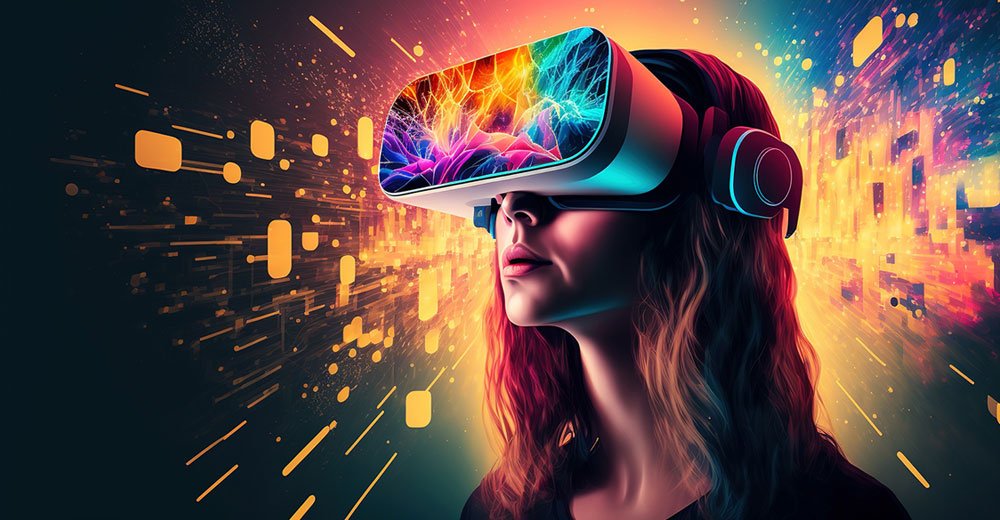
Featured Posts
-
Solve Nyt Strands Puzzle 422 Tuesday April 29th Hints And Answers
Apr 29, 2025 -
Reagan Airport Helicopter Plane Near Miss New Details On Pilot Negligence Emerge
Apr 29, 2025 -
Solve Nyt Strands Hints And Answers For March 3rd 2025
Apr 29, 2025 -
Post Covid Migration In Germany Impact Of Enhanced Border Security
Apr 29, 2025 -
Analyzing The Surge Temus Price Increases And The Legacy Of Trump Tariffs
Apr 29, 2025
Latest Posts
-
Trumps Potential Pardon Of Pete Rose A Look At The Mlb Betting Ban
Apr 29, 2025 -
Pete Rose And The Presidential Pardon Sports Betting And The Law
Apr 29, 2025 -
The Pete Rose Pardon Trumps Decision And The Future Of Mlb
Apr 29, 2025 -
Mlbs Pete Rose Ban Trumps Criticism And Promise Of A Posthumous Pardon
Apr 29, 2025 -
Will Trump Pardon Pete Rose Examining The Implications Of A Baseball Betting Ban
Apr 29, 2025